Generative AI Speech-to-Speech Technology: Current Trends and Future Prospects
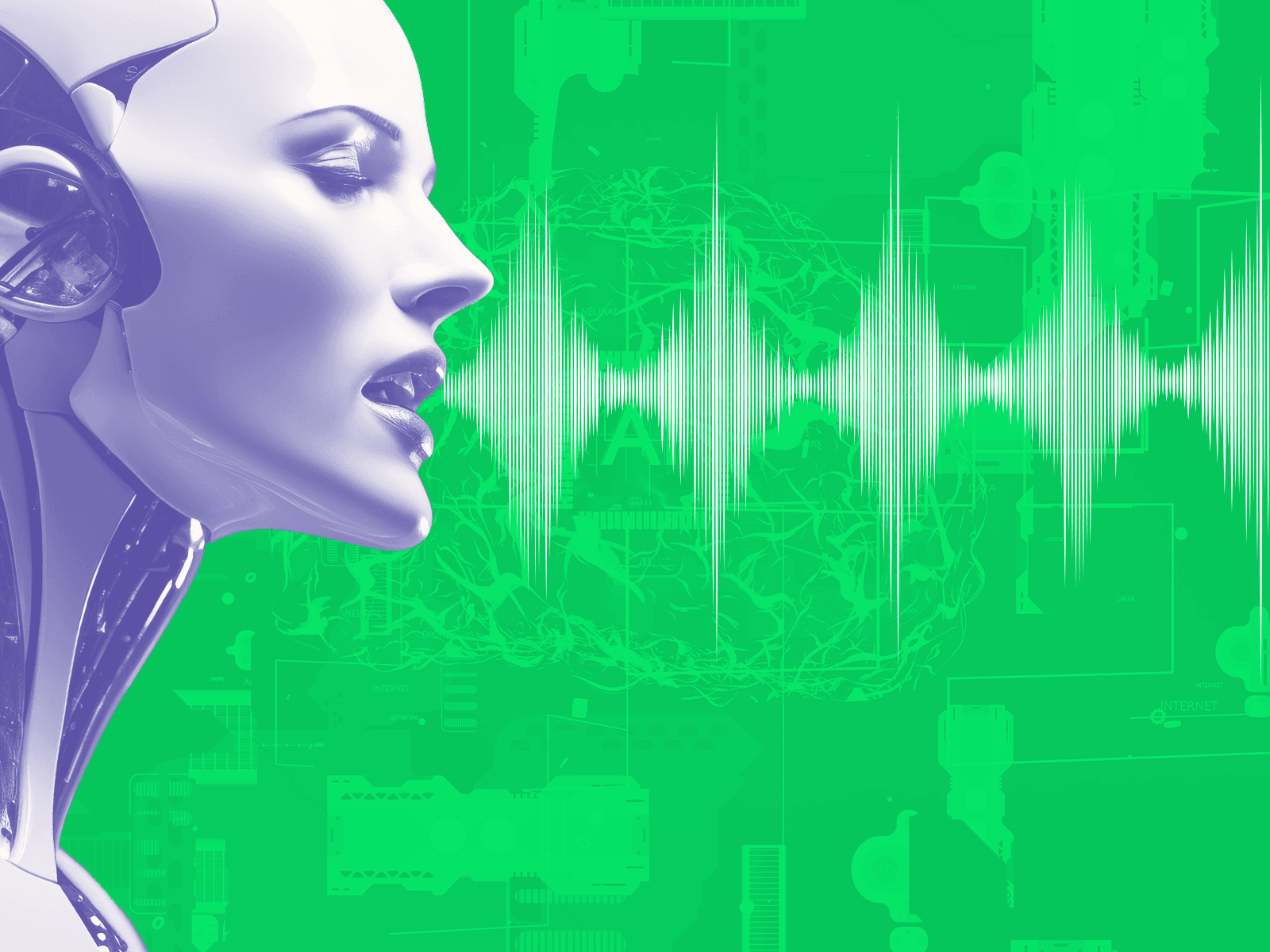
Illustration: © AI For All
Generative AI-powered voice technology is reshaping how we communicate, from language translation to accent augmentation, to complete transformation or obfuscation. This has created new opportunities for industries such as customer service, entertainment, law enforcement, and beyond.
As the technology matures, it promises far-reaching applications, but the journey is not without challenges, including scalability, quality, and ethics issues.
Let’s take a look at how AI-driven voice technology has evolved, the hurdles we face, and the potential it holds across different sectors.
How it Started to How It’s Going
The development of voice technology started with basic voice conversion systems that could modify vocal characteristics. Not surprisingly, these early efforts often produced unnatural, robotic-sounding outputs.
Thankfully, integrating neural networks and machine learning techniques has since revolutionized the field. Recurrent Neural Networks (RNNs) and Generative Adversarial Networks (GANs) introduced the ability to create more realistic voice transformations by capturing subtle vocal nuances like intonation and emotion.
In recent years, several breakthroughs have propelled speech-to-speech capabilities forward. Transformer-based models like OpenAI’s GPT-3 and Google’s T5, which excel in natural language generation, have been adapted for speech tasks.
These models leverage massive datasets of text and audio to produce more human-like voice augmentations that retain the original speaker's style and emotional tone.
This smoother and more coherent speech is vital for speech-to-speech tech to thrive in a production environment. Additionally, zero-shot voice conversion now allows the replication of a specific voice with minimal training data, which is a game-changer for a wide range of applications across industries.
Top Use Cases
Customer service and customer experience (CX) are areas where generative AI speech-to-speech technology has been particularly valuable.
Enterprises are using voice harmonization software that allows contact center agents to adjust their accents and tone in real time, ensuring better communication with customers.
Optimizing interactions and removing conversational barriers not only leads to a better experience for both agents and customers but significantly broadens the talent pool for companies looking to outsource off- or near-shore.
In the gaming and VR industries, AI speech-to-speech technology enables people to take on new personas, immersing themselves in a new environment, and modifying their voices for different characters or languages. It also offers a fun and innovative way to protect the identities of players when interacting with strangers online.
In that vein, in defense and law enforcement, voice technology not only enables officials to mask their identities but also clearly understand the person they’re speaking to in real-time. This is a critical component of acting quickly and effectively in time-sensitive, potentially dangerous scenarios.
Top Challenges
As most AI applications do, speech-to-speech technology raises some very real ethical concerns. For example, the potential misuse of AI to create deep fake audio that impersonates real individuals poses significant legal and security threats.
Additionally, AI models that neutralize accents or emotions, bring questions about cultural erasure and manipulation to light.
Bias is another tricky issue. AI models trained on biased datasets will replicate those biases in their speech outputs, leading to unfair or discriminatory results.
To address this, researchers are working to create more inclusive datasets and refine the algorithms to minimize unintended consequences.
Privacy is another growing concern, especially as companies collect increasing amounts of voice data. Protecting this data while ensuring transparency about how it's used is essential for maintaining public trust in AI applications.
What’s Next?
To improve the accuracy, efficiency, and security of these systems, the future is bright for AI-powered voice technology. New techniques in unsupervised and semi-supervised learning are likely to reduce the need for large, annotated datasets, making it easier to develop advanced voice models.
More sophisticated multi-modal AI systems that combine voice, text, and visual data to enhance context awareness and produce more natural interactions are another exciting area that will change the way we immerse ourselves in conversation.
While challenges remain, the potential of generative AI speech-to-speech technology far outweighs the risks. By striking a balance between innovation and ethics, we can ensure that speech-to-speech technology is used responsibly, inclusively, and effectively in years to come.
Generative AI
Natural Language Processing (NLP)
Author
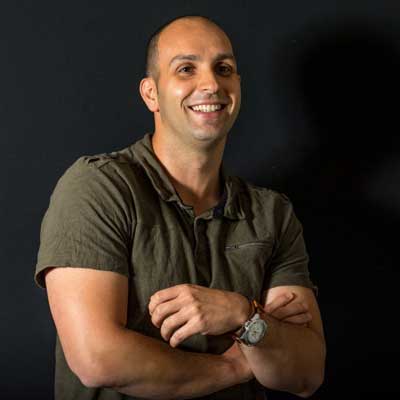
Yishay Carmiel is the founder and CEO of Meaning. He has a successful track record of building, launching, and growing disruptive, AI-driven, revenue-generating products and services across startups and Fortune 500 companies. The author of numerous research papers on conversational AI, machine intelligence, and deep learning, he's been recognized as a leading global expert in the field of voice technology.
Author
Yishay Carmiel is the founder and CEO of Meaning. He has a successful track record of building, launching, and growing disruptive, AI-driven, revenue-generating products and services across startups and Fortune 500 companies. The author of numerous research papers on conversational AI, machine intelligence, and deep learning, he's been recognized as a leading global expert in the field of voice technology.