Leveraging the Power of AI in Retail Operations
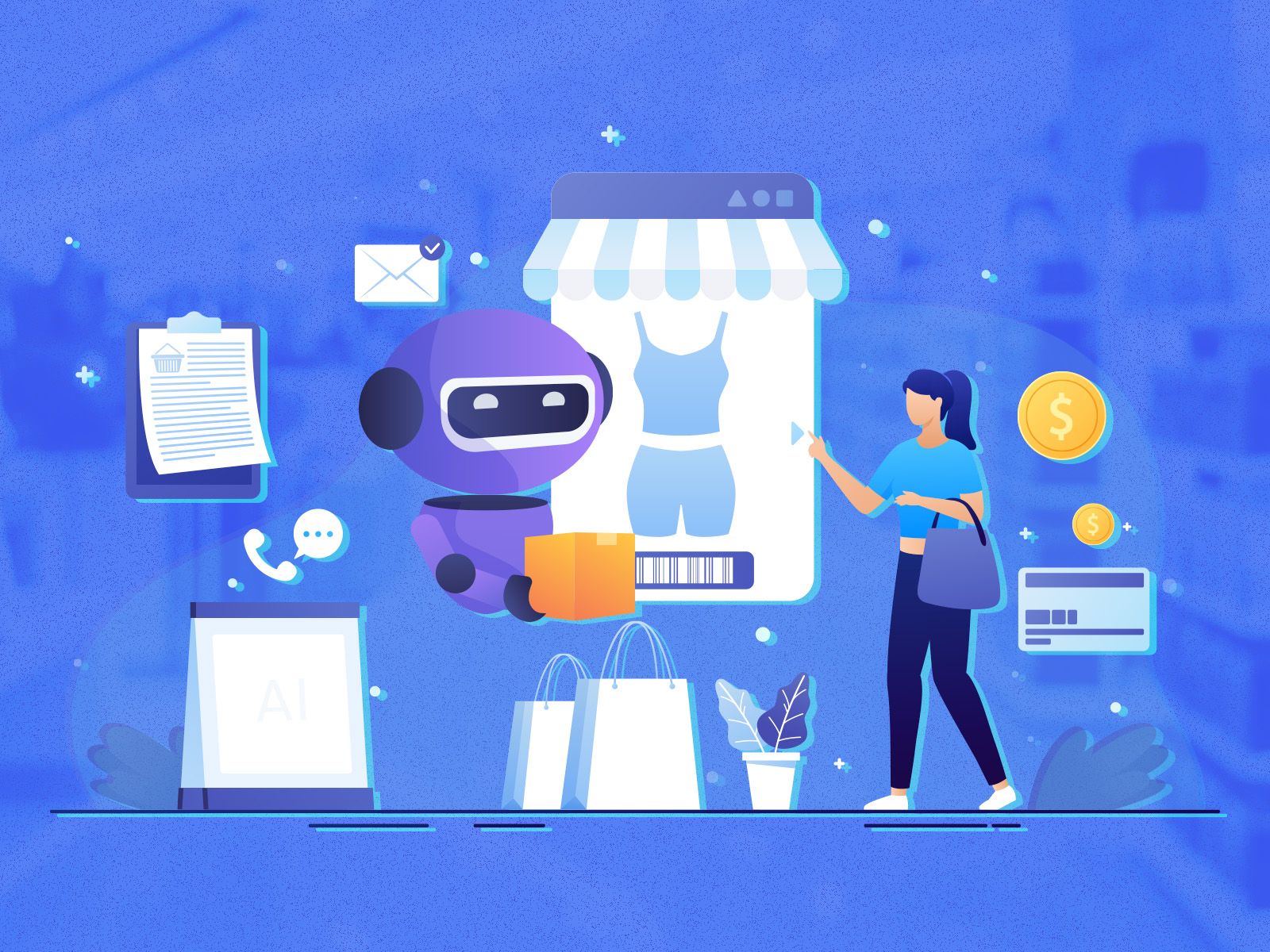
Illustration: © AI For All
Over the past five years of my career in data science and artificial intelligence, I’ve worked with several clients in different industries. Retail is one of my favorite verticals because AI has a great deal of potential for success in this area.
Retail businesses, as well as retail software providers, need to know about how AI works practically in the industry, as this allows them to improve workflows, gain an edge in the market, and increase sales.
Online Shopping: Virtual Assistants & Smart Recommendations
There has always been a great divide between virtual and physical storefronts. Each platform faces different customer service challenges, but it’s easy to see how shopping online can be frustrating for anyone with basic questions. It’s not as easy to just approach someone with a name tag and ask them a question.
You have to do research, scour product details, or even sit in a queue for customer support. In many cases, your customer support ticket might connect you with an agent who doesn’t know anything about the product you’re asking about.
Fortunately, advances in generative AI have made chatbots more feasible than ever before. In the past, businesses had to create rule-based algorithms with predefined flows to provide appropriate responses.
However, the rise of Natural Language Processing and GPT-based models has completely changed the game. If you are a chatbot with the right information, it can handle much more complex and humanlike customer service interactions.
Only as Strong as Your Weakest Data
No matter how advanced AI gets, data will always be at the forefront. The kind of data you need will depend on the kind of retail chatbot you’re developing.
You may need to train it with information like product descriptions, customer service transcripts between real humans, social media posts, and brand guidelines. The data you use must be relevant to the goal you’re trying to achieve, and quality should be at the forefront. You must go through the data and make sure it’s clean and free of any bias.
Product Recommendations
The powerful suggestion engines of Amazon and other online retailers are typically associated with significant investments. However, the same techniques are practical at smaller scales too.
The key to success is working out the features and architecture of the model to achieve your business goals. Besides, you should consider that people's behavior changes, and accordingly the model needs to be adapted to new realities, otherwise, it will be outdated and provide poor results.
There are a few different approaches that one can take to achieve a successful product recommendation engine:
- Collaborative filtering: Leverages past customer behavior and preferences to suggest items.
- Content-based filtering: Utilizes item characteristics and features to suggest comparable items.
- Social filtering: You could use information about users to find other products that other shoppers in their segment have left positive ratings for.
These are just a few strategies that you can use, but the best strategies will be hybrid approaches — using more than one method to suggest products to users. Deep learning can take this to a whole new level, making it possible to dive into complex patterns from large datasets to make more accurate suggestions.
Leveraging POS Data Analytics
AI isn’t just getting stronger in its customer interactions. It’s continuing to cement its mastery over backend data analysis and forecasting.
Your POS system is one of your most powerful data generation tools as a retail business. The amount of data generated can be overwhelming for humans, but this is the arena where AI shines the brightest.
Machine learning forecasting models allow businesses to aggregate their historical data, revealing patterns that can predict demand in the future. With robust data collection channels, businesses can develop automated pipelines that report regular demand forecasts.
AI-powered demand forecasting is one of the most promising POS system features, especially if you want to get a competitive edge. However, you need to implement it right to get the outcomes you’re expecting.
The Demand Forecasting Process
The time it will take for your business to get started is directly related to your standards for accuracy and specific goals. Demand forecasting utilizes machine learning forecasting models that analyze historical data, identifying patterns and making predictions about future demand.
This process involves incorporating sales data and various other relevant information from previous periods. These insights can then be integrated into an automated pipeline, generating demand forecasts regularly, whether on a weekly, monthly, or quarterly basis.
Demand forecasting isn’t the only thing that AI can predict. You can also predict future sales, and not just for the entire business. Sales forecasting can reveal future trends for specific products.
Through these insights, you can track the performance of your lowest-selling products and gain valuable information about how to respond. It’s important to measure actual sales and demand and compare it to the forecasted values to further train the AI model.
Develop Marketing Strategies Supported by AI
Another opportunity that AI provides for the retail industry, is its potential to enrich marketing efforts. AI makes the ideation process much more efficient, and in many cases, marketers can automate entire content creation processes with generative AI tools.
However, AI is a double-edged sword. Although AI tools are more effective than ever before at content creation, they lack human understanding and empathy. AI is much better suited as a tool to enhance human marketers rather than a replacement for marketers outright.
Speeding up the process of creating article titles, doing research, keyword generation, and topic clustering means more time spent on writing the actual content, which meets your marketing objectives.
AI can also make marketing processes much more personalized at scale. Segmented emails, automatic review responses, and product recommendations are just a few of the possibilities.
Achieving Retail Success with AI
Whether AI in your business is customer-facing, backend-focused, or both, it’s important to first take a step back and understand your goals, needs, and the state of your business.
Consulting with AI and data science experts is a fantastic place to start to find the best market fit for a new AI product. They can also help you develop effective and affordable strategies to incorporate AI into your business.
Retail
AI Assistants
AI for Business
Author