Exploring AI's Tricky Side: Bias and Fairness in Healthcare
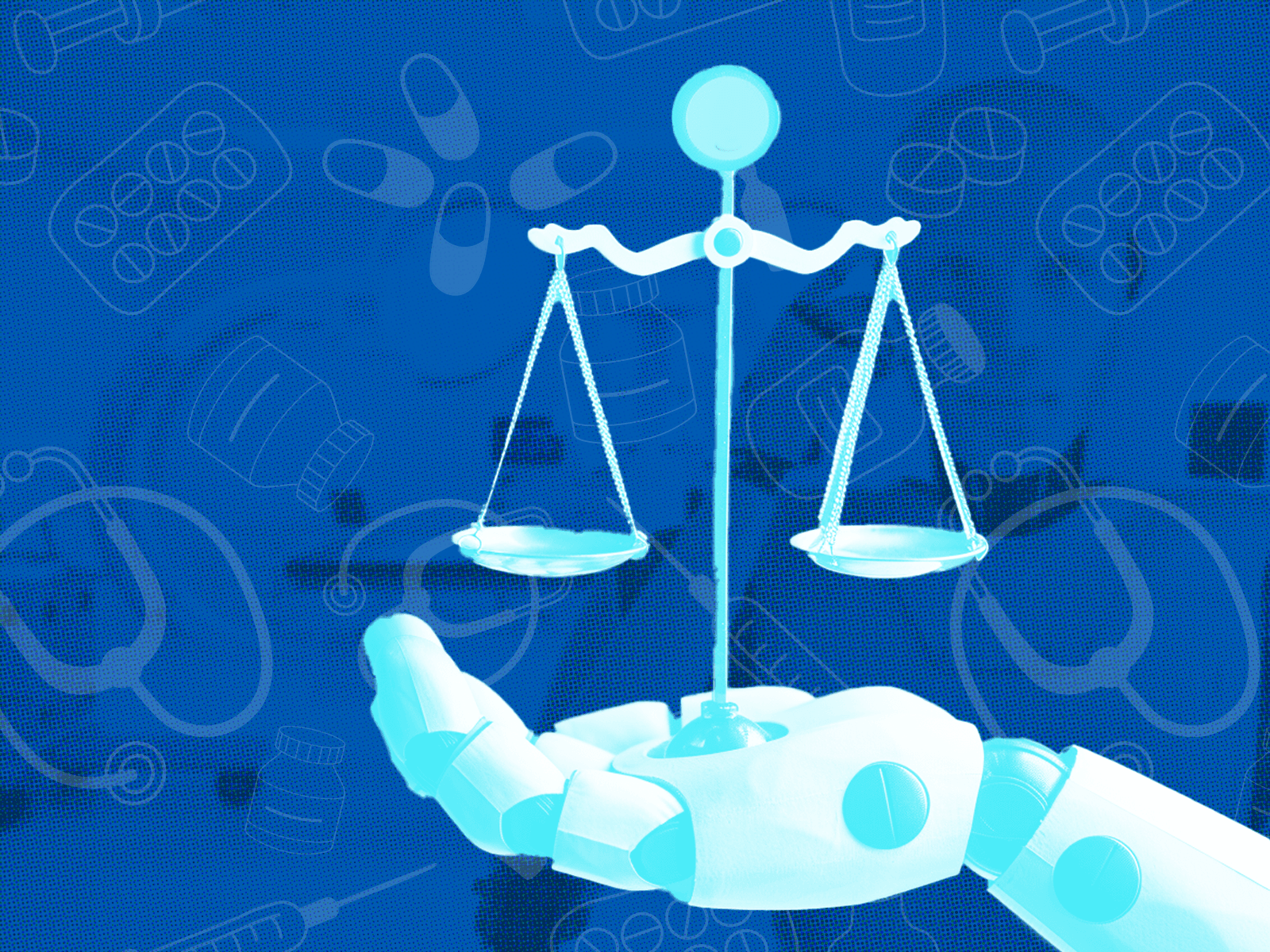
Illustration: © AI For All
Artificial Intelligence (AI) has achieved everything from streamlining simple human tasks, to making massive impacts in the enterprise. But it also has a blind spot—it can pick up our human biases. So, as AI becomes increasingly intertwined with our daily lives, it begs the question: How honest are our AI companions? Are they telling us what’s accurate, or simply what we want to hear?
From talent acquisition, to accelerating clinical trials in healthcare, and summarizing complex legal documents, the AI systems we're building are sometimes playing favorites, and not in a good way. When we teach AI to think like us, not only does it pick up our intelligence, but also preferences, prejudices, and other flaws. After all, we’re only human.
But the biased algorithms we teach don't just stay in the computer—they spill into real life, which can have major implications for certain people, processes, and decisions. This can span gender, racial or ethnic, and social factors. And for these reasons, one industry we need to be especially cognizant of bias in is healthcare.
It’s important to note that biases are not always negative. In fact, a certain degree of bias is essential for models to make accurate predictions and decisions based on patterns within the data they’ve been trained on. Without bias, AI models would cease to understand complex language patterns, hindering their ability to provide accurate insights. The real problem arises when the bias becomes unfair, unjust, or discriminatory.
So how do we get AI to play fair? It’s not an easy question, as bias in language models mirrors the bigger problems of inequality in our society. Let’s look at several problematic areas of bias in our healthcare system and beyond, and how we can address them to even the playing field and make a more equitable AI experience for all.
Gender Bias
When AI models generalize from biased data, they’re more prone to making incorrect or harmful assumptions. For example, consider a natural language processing (NLP) model trained on historical data that suggests only men can be doctors and only women can be nurses. If the model recommends only men pursue their doctorate, unfair gender stereotypes are perpetuated and career opportunities become limited based on gender.
Unfortunately, the fix is not as simple as changing “he” to “she” in a sentence. For many years, the field of medicine has been male-dominated, and the gender disparities in the representation of doctors persists today. Therefore, when the sentence is gender-neutral, it fails to acknowledge real-world gender imbalances within the profession.
In this scenario, and many others, gender bias in language generation is not about explicit discrimination, but rather manifests through subtle inequalities and nuances in society. To address this, it’s crucial to improve the data quality by including diverse perspectives and avoiding stereotypes. Of course, this is easier said than done.
Social and Cultural Bias
Similar to gender bias, ethnic, racial, and religious bias is a major concern for AI users. Language models have been found to produce racially biased language and often struggle with dialects and accents that are not well-represented in their training data. This can lead to harmful consequences, including reinforcing racial stereotypes and discrimination, ultimately preventing people from seeking or being able to obtain appropriate care.
Economic biases in AI are another area often overlooked, but that can be equally detrimental. AI systems may inadvertently favor certain economic classes, making it harder for those from disadvantaged backgrounds to access resources and opportunities. This is one of many social determinants that can put those with affluent backgrounds on a pedestal.
To mitigate this, it’s essential to diversify the training data, include underrepresented groups, and employ techniques like adversarial training to reduce biased language generation. AI developers should consider the potential impact of their models on different social and cultural communities—especially when considering diverse patient populations.
Sycophancy: Appeasement vs. Authenticity
Sycophantic behavior refers to a tendency to flatter, agree with, or excessively praise someone in authority or power to maintain a harmonious relationship or gain favor. Essentially, it involves echoing the opinions or beliefs of others, even when those opinions are not grounded in truth or aligned with one’s values or thoughts.
In AI, sycophantic behavior becomes problematic when these systems prioritize telling users what they want to hear rather than providing objective or truthful responses. This can support misinformation and limit the potential of AI to provide valuable insights and diverse perspectives. Sycophancy is more likely to occur when AI is posed with questions on topics without definitive answers, such as politics vs. mathematics.
Recognizing and addressing sycophantic behavior is crucial in fostering transparency, trustworthiness, and authenticity in AI, ultimately benefiting users and society as a whole. It’s essential to consider the model’s performance critically and fine-tune it to improve its accuracy, especially in domains like healthcare where precision is crucial.
As new regulations around responsible and ethical AI come to light, much of the focus is spent on security implications and doomsday scenarios. However, most fall short on the very practical, yet problematic question of whether or not AI systems are biased or fair. As AI becomes a greater part of our personal and professional lives, much consideration should be given to these areas.
Healthcare
AI Bias
Author
Author
David Talby, PhD, MBA, is the CTO of John Snow Labs. He has spent his career making AI, big data, and data science solve real-world problems in healthcare, life science, and related fields.