On this episode of the AI For All Podcast, Jean-Simon Venne, CTO and co-founder of BrainBox AI, joins Ryan Chacon and Neil Sahota to discuss the current state of enterprise AI adoption and self-operating AI buildings. They talk about how enterprises are viewing AI, using AI for energy optimization, the benefits of AI smart buildings, AI safety, the process for implementing AI in a building, overcoming fear of AI, the global impact of AI, and the exciting future of AI.
About Jean-Simon Venne
Jean-Simon Venne is a co-founder and CTO of BrainBox AI. As a technology expert specializing in the fast and efficient migration of technological innovations to commercial applications, Jean-Simon has over 25 years of experience developing and implementing new technology to solve long-standing commercial issues in the fields of telecommunications, biotechnology, and energy efficiency. Prior to joining BrainBox AI, he was responsible for the successful integration of M2M technology in over 200 smart buildings across North America, Europe, and the Middle East.
Interested in connecting with Jean-Simon? Reach out on LinkedIn!
About BrainBox AI
BrainBox AI uses deep learning, cloud-based computing, algorithms, and proprietary processes to support 24/7 self-operating buildings that require no human intervention and enable maximum energy efficiency. BrainBox AI enables a reduction in total energy costs of up to 25% in less than three months, with low to no CAPEX needed from property owners. It also improves occupant comfort by 60% and decreases the carbon footprint of a building by 20-40%.
Key Questions and Topics from This Episode:
(00:39) Introduction to Jean-Simon Venne and BrainBox AI
(01:10) Current state of enterprise AI adoption
(03:09) How are enterprises viewing AI?
(05:17) Asset management and optimization
(06:58) Balancing the use of energy
(08:33) AI in smart buildings and the benefits
(11:46) How is AI helping reach sustainability goals?
(13:54) Configuring an AI smart building
(14:50) AI safety in smart buildings
(16:34) Implementing AI in a building
(18:22) How building data is handled
(19:27) What needs to happen to increase adoption?
(21:52) Overcoming fear of AI
(25:38) Global impact of AI
(29:48) What's exciting about AI
(32:12) Learn more about BrainBox AI
(01:10) Current state of enterprise AI adoption
(03:09) How are enterprises viewing AI?
(05:17) Asset management and optimization
(06:58) Balancing the use of energy
(08:33) AI in smart buildings and the benefits
(11:46) How is AI helping reach sustainability goals?
(13:54) Configuring an AI smart building
(14:50) AI safety in smart buildings
(16:34) Implementing AI in a building
(18:22) How building data is handled
(19:27) What needs to happen to increase adoption?
(21:52) Overcoming fear of AI
(25:38) Global impact of AI
(29:48) What's exciting about AI
(32:12) Learn more about BrainBox AI
Transcript:
- [Ryan] Welcome everybody to another episode of the AI For All Podcast. I'm Ryan Chacon and with me as always is my co-host Neil Sahota, the AI Advisor to the UN and founder of AI for Good. Neil, how's it going this week?
- [Neil] I'm doing all right. I hope everybody out there is having an awesome week so far.
- [Ryan] We also have Nikolai, our producer, who's going to be listening in and chiming in some good questions throughout. Nikolai, how's it going?
- [Nikolai] Going good. Hello, everyone.
- [Ryan] All right, on today's episode very exciting conversation planned. So, we are going to be discussing the current state of AI adoption in enterprises and what needs to happen to really grow the reputation of AI in the eyes of companies and that enterprise space.
To discuss this, we have Jean-Simon Venne, the Chief Technology Officer and co-founder of BrainBox AI. They are a company that is focused on using deep learning, cloud based computing algorithms and proprietary process to support 24/7 self operating buildings that require no human interaction at all and enables maximum energy efficiency, which we'll definitely dive into here in a second.
Jean-Simon, thank you for being on the podcast.
- [Jean-Simon] Hey, thank you for inviting me.
- [Ryan] So, let's kick this off. I want to ask a kind of high level question to start this conversation. From your perspective, how do you view the current state of AI adoption within enterprises?
- [Jean-Simon] It used to be fairly slow, I would say, until last winter. And then suddenly we had a huge jump where, thanks to GPT model that was released in November, then it opened the floodgate. We're seeing so many new application that are geared toward businesses. We still see a lot of applications geared to consumer, as everybody knows, being released every week. But at the same time, there's a load happening on the commercial side, on the enterprise side, which are all geared toward improving productivity, I would say, at all level, right? And it's really overwhelming right now. Of course, some of these applications will be a firefly. They will have a lifespan of a few weeks, then they will disappear. But some of them will percolate to the top and will become the blockbuster used by most of the company and will have an impact.
I'm convinced now, like looking into it for the last six months, how it's progressing. It's probably more, one of the most fast paced, high tech revolution that we're going through right now that we ever seen, right? It's going much faster than the Internet in the nineties. It's going much faster in terms of the speed of penetration rate that we've seen with the different intelligent phones.
And it's just very overwhelming when you're trying just to stay on top of it. Try to figure out like what's happening, what's going to be these blockbuster, what's going to be these firefly and where all this is going. So trying to predict right now where we're going to end up in October or November is extremely hard because it's just moving, it's overwhelmingly fast, right?
- [Ryan] Are most enterprises viewing AI as a good thing or are there organizations and enterprises, maybe it's based on what they do, size, industry, you name it, that are maybe hesitant or don't view AI as maybe as positive as many of us do.
- [Jean-Simon] All depending on what are you using AI for, right? So if you use AI to resolve the air traffic control congestion that we're going through right now, I guess for most people inside the enterprise or outside the enterprise, a very good thing, right? So as the weather is becoming very complex to manage, it's becoming a nightmare to manage the air traffic control in any busy area like New York, Chicago, Atlanta, and do that in real time while you have these supercell going up and down everywhere on the weather side.
And you have these thousands of plane in the air and you need to figure out how you're going to manage your time slot for landing and departure. If you don't have now these AI tool, you're just going to not going to make it. You used to make it through an excel sheet and doing a good planning. Now it's not possible anymore, and you need these tools.
So it's a very good thing. Where it's starting to be quite interesting is to use these tool which are very good to do prediction so and to take that prediction, which is coming out of these deep learning model, and use that prediction to change the way you're managing your asset and think of whatever type of asset, it could be plane, boat, car, or building.
And how do you optimize these assets, which cost you a fortune to build, and are still costing you a fortune to operate on a daily basis? So how do you use these assets, and how do you optimize their usage? So you could operate them at a lower cost or get more productivity out of them.
And that's where there's a huge space opening up in that area, which is really helping big corporation and small corporation to do a better job at a lower cost on a daily basis.
- [Neil] Talking about infrastructure then, right? You're talking about asset management, which is often a neglected area for business, just expect things to work.
How can we or how is like BrainBox helping to improve that?
- [Jean-Simon] We're focusing on a very limited space, of that huge space of asset optimization which is the commercial building and the energy, which are consumed by these commercial building on a daily basis. And you're right like Neil, it's something we tend to forget, right? You're operating all of the hospitals, these schools, these office high rise, these airport, and it used to cost you whatever in energy, and it is what it is, right? So why should I revisit that topic? It is what it is. And the suddenly if you start to use AI to optimize the energy consumed by these buildings, so you're using that prediction, and you are capable to lower the energy consumed by these assets, that's going directly to the bottom line. If you're operating an hospital, and you're reducing your energy budget to operate that hospital, it goes directly to your bottom line in terms of making a more efficient operation on the financial side of it. So the same goes to whatever type of building, commercial building you're operating. And it was quite interesting is not only you're satisfying the CFO by having a better control on the budget, but at the same time you're reducing the emission, so you're having one stone, and you're basically hitting two targets at the same time, which is lowering my expense to run that asset. At the same time, I'm reducing the emission, giving a break on the impact of that building on the planet.
- [Neil] Is there a balance or a trade off? Because if you're using more AI, then you're using more computing power, so in theory, you're using more energy than HVAC.
- [Jean-Simon] Yeah, it's a typical question that everybody asked. So are you not transferring saving kilowatt at the building level for heating and cooling at the same time increasing kilowatt in the cloud for training these model? Absolutely. So the good thing about playing with numbers when you're doing deep learning, percentage on off these small number is the crunching, the training energy that is required for the neural net are much slower than if you're playing with image or facial recognition, movie, and it's fascinating to see how little it would cost to train a model. So to give you an example, when we train like a typical high rise data set from a building in downtown Manhattan, it will take about an hour of computing to have a full training of that AI model, which is literally impossible if you're training it on facial recognition type of architecture, which is much bigger computing power to get any type of training.
So the good thing is it's not that bad, but yes, we're monitoring it and we're offsetting whatever we're saving at the billing level because the total equation need to be like a very compelling equation when you're factoring both consumption together.
- [Ryan] Let me ask you, this is actually a topic we haven't dove into before is really talking about how AI is being used in buildings or in smart buildings. Can you just break that down for audience who may not be as familiar or maybe be interested in kind of understanding if they're, they own buildings or property and how AI can be incorporated in and the benefits that it's going to provide them.
- [Jean-Simon] Yeah, absolutely. And I'm going to give you like a parallel with a spaceship because usually that people understand that phenomenon of trajectory in spaceship because we've all seen it in so many movies over the years.
So what's happening in a building is all of the commercial building today, they are operating on a programmation, so on a coding, so on a control sequence that you program into your building and your schedule and the different equipment operate onto the same way that it's operating into your living room.
So in your living room when the thermostat has been asked to the deliver 72, you will not, the thermostat will not react until the temperature deviate from that 72. So as the temperature started to decrease because it's in the wintertime, and it's getting a bit colder in your living room, the thermostat will say I'm 71, I'm going to trigger eating because I want to go back to my 72. And it's going to do that operation of reacting to something that is already happening in your living room to correct the situation and bring it back to the desired temperature. So in all of the big buildings, think of hospital, hotel, commercial building, office tower, it's the same way.
It works exactly the same way. It's trying to maintain and react to things that are happening to make a correction and bring you back to what you want to be in terms of temperature and humidity level. When you're using deep learning where you're getting the prediction, so we're training, we're taking all of that data, and we're training model that are telling us what will be happening in your living room in the next six, seven hours.
So we know that in two hours in your living room, it will be too hot or too cold by two degree or by one degree. And we know exactly what will be happening to you. Knowing that, we then run all of the possible control combination that we could run to change that future for a better future in terms of energy spending.
So it's a bit the same than in a spacecraft which is going off trajectory, and it will need to consume fuel to go back on trajectory. But if you know in advance that you're going to go off trajectory, you can make a small fuel consumption just to prevent that off trajectory course from happening.
And then you stay on trajectory, and it costs you a lot less fuel to stay on trajectory because you knew in advance what will be happening. So, I also give this other example of it's a bit like in the movie Back to the Future. We have the capability to go in the future, see how horrible it is, then we come back in the present. We make a few adjustment, and we change that future for a better future. So, it's exactly what we're doing on the heating, cooling, and ventilation of the building, and that's where most of the energy goes in a typical commercial building.
- [Ryan] Bringing in AI is going to help a lot of these organizations meet sustainability goals that they have. I know that's a big thing for a lot of organizations now is how they're incorporating or what their sustainability goals are and how they're achieving them. We talked about this on our other podcast more focused on IoT and bringing in sensors to be able to detect and better monitor buildings, making them smart buildings.
But on the AI side, how is that kind of playing into the sustainability goals organizations have?
- [Jean-Simon] Thanks to the video game industry, we take the architecture that they already built, which is how do you win a video game, there's several objectives that you have to fill in and depending on how much point or bonus you're going to get for these objectives, you're going to win the game, and try not to get killed then and because that's not a good ending. So these multi objective, that's exactly what we basically move into our algorithm, and we say, okay, to win the game because they, I really like to win. And so how do you win the game? You need to lower the energy, but you also need to maximize the number of emission reduction you're going to bring to the table. And that's that multi objective. This is where you basically get the full power of AI because it tries to basically get both at the same time. He's trying to say I'm going to lower that money, I'm going to same time try to optimize my emission reduction. And it's funny to see because we see in the building, which are using natural gas, let's say, to do heating versus electricity, so they might use electricity to do the cooling and then they might use natural gas for the eating during the wintertime.
We see that the AI is saying, wait a sec, when I reduce my natural gas, I get more bonus because I not only save money, but I save a lot more emission than when I reduce electricity because electricity is produced by a windmill in this area and really, I'm not gonna have a big impact on the emission if I reduce my electricity.
So right away, you see the AI trying to optimize that equation in a very specific building, depending how the energy source is coming into that building. And it's quite fascinating to see how performant it is.
- [Neil] What then governs the optimization rules? Is this something that's like standardized or is it configurable by each enterprise?
- [Jean-Simon] Yeah, it's an excellent question. Right now we're locking it at equal weight. So you're, you save money, you save emission, and it's equally weighted, but we have a lot of customers which are asking us can I play with these weighted factors, and I want, I don't care about money, I just want to have a emission, and there's other customers that might say I don't care about the emission, I just want to save money because this is how my bonuses is decided at the end of the year as a manager of the asset, right? But then you can start to see how it could be twisted by objective, which are really personal to that human managing that asset. So, it's opening the discussion to a more ethical and philosophic questioning. But yeah, absolutely, it's something that could be done.
- [Neil] Does that mean that to some degree we have to bake in some safeguards? If it's a major heat wave, and you're, the AI is focused on just cost savings, would it necessarily or unnecessarily shut down some of the HVAC in some of the rooms, even if there's a bunch of people in there.
- [Jean-Simon] Excellent question, Neil. And actually when you play with AI, you have to put these, what I call these rules, these safety rules in place because if you don't put these boundary, you might have some extreme conclusion which would not consider some very important factor like the well being of a human. So absolutely. So, we have these rules that basically will make sure that the AI stay in a sandbox, and if you try to implement a scenario which is violating these rules, we're blocking you right or and that's all of the output of the AI, it goes through these filters of rules, and if you're violating one of the rules, we're not going to let you right back to that pump or that ventilator or that boiler because the scenario you're trying to follow is not acceptable. So, we keep adding these rule as we go forward. They're basically creating constraint, but at the same time, they're becoming the safeguard.
We actually building independent set of rules, and we need to want, we want to have unanimity in between these different set before letting anything being written back to the different controller and of a given building.
- [Nikolai] So for an enterprise or enterprises that would like to implement this technology, AI in their building, what does that process look like? Can any building get this technology?
- [Jean-Simon] There's all kind of building out there. I think the last time we checked, there was over 700 control protocols for heating and cooling and commercial building on our marvelous planet. Some of these protocol, the company that created them does not even exist anymore, but the protocol is still running in the building.
So you need to have a protocol which is not too old, that is still supported, that we still could connect to because that's is how we're connecting ourselves. We're connecting ourselves to the existing control system of the building, so it needs to be compatible, right? So we are not capable to offer compatibility with everything that exists out there.
We try to cover about 80 percent of the pie. And once we connect to that control system, we start to extract that data in a read only fashion for about five to six weeks because we need to train these neural net, right? They need to be trained to become able to give us that prediction quality.
So that period of time is something we could not really go around. So you have to wait for that training to happen. And once that is completed, and we reach a prediction quality, which is good enough to be able to start to do the control, then we start. So that's pretty much how the process goes.
There is also a process that we do on our side, which is to clean your data, to map your data, and to tag your data. So, we enrich the data to a level where everything is map and tag, because that's, that is what the different algorithm need to understand what are they dealing with. So, that's something we do internally. And when it's done, we're usually good unless you keep extending your building or constantly making new wings to your building or adding more feature to your building.
- [Nikolai] Do you augment the data in any way?
- [Jean-Simon] We don't really augment the data. We will do gap filling. So sometimes these controllers, they don't give you the value anymore for let's say 5, 10, 20 minutes. We will fill the gap. So we do synthetic data gap filling because neural net algorithm, they really don't like gap. They tend to become very annoyed by it. So, we like to make it, if it's longer than 20 minutes, we will stop doing it, and we're just going to release the building. But, we do augment the data in a sense that we're going to have to detail weather for that specific location that we're buying from the different company which are selling us that data.
And when I'm talking detail weather, I'm talking about wind direction, wind speed, wind gust, thickness of the cloud at any given time around that location, which is the same thing that the airplane pilots are using to figure out where they should go around and fly through their flight path.
- [Ryan] In order for us to get to that next level of adoption for enterprises, whether it's in the smart buildings, real estate space, agriculture, industrial, you name it, what do you think really has to happen for us to get to that next level? Is there, are there hesitations that need to be worked through that organizations have? Is there a global event that AI has to come in and contribute and solve and change and really become the headline? What do you think it is that's going to get us to that next step?
- [Jean-Simon] My answer would have been completely different if it was like 10 months ago. I would have said yes, we need it. I think it's happening right now. I don't think we need that event anymore. It's happening, and it's happening extremely fast as we speak.
And I think most humans really don't understand how fast things are going right now, and they're being completely blindsided and where there's going to be a wake up moment. So it's not like an event that's going to trigger reaction. I think there's going to be, the reaction is going to be the oppose it when we, most of the people will realize how fast it's going, and then there's probably going to be a scare moment, right? They're going to be like, whoa, wow, should we, and then again, I think we're reaching the point here where are we ready to have that much power because there's a lot of power that is being democratized and unleashed right now.
And the question is, what are we going to do with that power? I said in a conference about two months ago, I said, we're facing the greatest challenge we ever had as a human race, right? The global warming is probably our biggest challenge that is ahead of us. We will overcome it but we need help.
And at the same time that we're facing that huge challenge, we have this incredible power that is given to us through the AI advancement that could give us a lot of tools to resolve that challenge. So there's, we're lucky, right? We have the challenge, but at the same time we, we received this tool, which is extremely powerful, and we could do a lot of things with it very fast. So now it's just a matter to be wise and not stupid and to use it in the right way. So it's all dependent on us at this point.
- [Neil] I love the, the way you're framing this, that this could all be an opportunity to help us solve big problems like climate change. But you, I think you also touched upon something that unfortunately is a big challenge for us.
There's a lot of rapid change going on. I think people are starting to realize that. If you look at the Writer's Guild strike, for example, it happened a little earlier than people expected. Some of the visceral nature of some of the demands was, I think, caught people off guard.
Particularly, they don't, they're, one of their things is they don't want any kind of use of AI in making films. And I think one of the reasons this got triggered was we saw ChatGPT go from 10,000 users to 100 million in a month, and suddenly it was real, right? And rather than look at this as a tool, an opportunity where the writers could produce more scripts, maybe improve some of the quality of scripts that, again, human and machine working together, they saw it as something to be afraid of.
How do we overcome that, right? Because I agree with you, this is just a tool. There's a lot of opportunity, but I think a lot of people struggle. We're wired to see threats. How do people unlock the opportunities?
- [Jean-Simon] I think that's what's keeping me up at night. It's, where I'm worrying is when I see things like I saw last week. I don't know if you saw that news update that there's a startup that, I'm not going to give the name, but the startup that have these girlfriend, boyfriend friends based on the LLM models. They already have 10 million downloads and 250,000 paying premium package in force.
And the people are saying that it's actually better than my girlfriend. And you hear that, and you go like, as a human race, we're in trouble. Because there's a level of lack of maturity on how to use these tools and what are you trying to do with these tools? So, it's the pace that it's moving at, it's a bit worrisome because it's already more powerful than us in terms of knowledge acquisition, right? So it's capable to acquire so much knowledge that we can compete with it. And it's capable to transfer that knowledge to another model in a matter of hours, which us human, we're totally incapable of doing, right?
So, it's very already outpacing us. And the question is, what are we going to use it for? And that's where we need to figure that out very quickly because it could turn out in a very bad way. I tend to stay optimistic in a sense that if we show the way of how it could be used, either for air traffic control or to reduce the number of boats on the ocean to transfer, to move at the same quantity of cargo or to lower by 25 percent the energy being spent by all of the office tower in Manhattan, that's going to make a big change, and that's going to pivot into a better world, right? So I'm not so much of a, of the girlfriend model. I'm more a fan of the let's use it the right way. And I don't know if I answered your question, but it's, we need to have more people showing the way of how to do it right.
- [Ryan] Where do you think as we move forward over the next, maybe six months is too short of a period, but like next year or so AI is going to have the biggest impact globally. And Neil, I'd be curious to hear your opinion too, with everything that you're doing with AI for Good, what you all have been talking about, where you see AI as having the biggest global impact.
- [Jean-Simon] I'm going to talk on the energy side of the, of that question, and then maybe I'm just going to add something on the productivity side.
So on the energy side, I think we're going to very quickly see AI adoption not only at the building level, so the demand level, but also at the grid level, because the grids are facing a complete nightmare in terms of managing because they're, they used to produce energy, transport the energy, and serve the demand. Now, they're facing like a complete puzzle where there is all kind of production which are not stable because sometimes there's wind, sometimes there's no wind, sometimes there's sun, no sun, and they need to manage that with a demand which is growing and at the same time now there's battery, and we need to modify, flip the transportation industry on the electricity grid by charging the electric vehicles.
It's becoming very complex for them to manage, and the AI will give them the tool to basically manage that ecosystem with a prediction capability, which is going to give them the ability to manage the domain in real time very efficiently. So think of California, but think of the eastern seaboard, these areas are in great need for that.
So we think we're going to start to see that happening within the next year very rapidly. And of course it's going to, it's going to include, how do you modulate the demand in that ecosystem in real time to maybe it's not the time to cool down the office, maybe it's time to charge the electrical vehicle, which are in the garage in the basement, and we're going to go back to the cooling in an hour.
So that kind of modulation which is really cannot be done by hand in an Excel sheet by human at this point. It's way too complex. On the productivity side, we're already seeing the impact, right? And that's what we have to manage. AI will replace jobs in all of the corporation and how do we manage it, so it's not becoming a social disruption that will create all kind of problem, not only on the economic side, but also on the political side, right?
- [Ryan] Neil, what about from your perspective? What have you all been talking about at the UN or what have you seen from the conversations that you've been having?
- [Neil] The simple answer is it's AI for good, that across the 17 Sustainable Development Goals, that's the focus. Actually, at the summit this year, it was actually one of the things that was announced was AI for Health. So you have the General Assembly, AI for Good which is housed in the ITU, which is the IT agency for the UN, the World Health Organization, WIPO, which is actually the World, I think, IP, Intellectual Property Organization, actually now partnering together, and they're about to activate a bunch of projects just on AI and healthcare.
You're going to see a similar thing for AI for finance because financial inclusion, financial literacy is one. So if you're going to look at the 17 SDGs that cover the major facets of like society and wellbeing and the planet, I think that's where you're going to want to see all these things.
This ecosystem to do good is, I think, thankfully much larger than most people may realize, but we still need more people actively involved and have the mindset of thinking that way. And I think, as Jean-Simon has been talking about, we're making that, I think, starting to make that transition, it's just that we as humans don't quite learn as fast as the machine, so it's, we're a little slower on the uptake.
- [Ryan] Jean-Simon, before we let you go here, you already mentioned what keeps you up at night, but looking at the other side of that, what excites you the most about where the future of this technology when it comes to AI solutions and tools being utilized in the enterprise space is going? Where, what are you most looking forward to? What gets you excited as opposed to what keeps you up at night?
- [Jean-Simon] I'm not that young. All my career, I've been playing with technology, and I was always frustrated for two reasons. It's either because it was not possible to do. The technology was not there yet or because the CFO was saying it's too expensive. You're not going to have the budget. So now these two things are gone.
We're opening a kind of a sandbox now where the technology is so powerful, and the cost is really not that high, that we could start to assemble all kind of new innovation technology solution, and we're just limited by our capacity to brainstorm and create them. And this is really the first time that it's happening when you think about it.
There's suddenly, it's a, the limitation is us being able to imagine the solution and putting it together. That's the limiting factor. So that's extremely exciting. You want to create a new protein. Go ahead. You want to create a new antibiotic. Go ahead. You want to find a solution for cancer. Go ahead. You have the power to find it now. You're limited by your imagination on how to assemble the different pieces together to find a solution. Let's start to be creative and make it happen.
- [Ryan] What you're most excited about, what we're most excited about, it's just the whole thing, the whole industry right now is there's a lot of optimism around it, and I think that's what excites me the most is that people are really starting to pay attention. They're starting to see the positive impacts. I feel like for many years, we've been in a situation where anytime you bring up AI, there's that battle of what it can do from a good perspective versus, oh, it's going to take jobs, it's going to take over the world. It's going to do all these negative things. And it's like well we're starting to see the actual applications now with a lot of these new tools and solutions in the, in our daily lives, not just on a personal level but also in our workplace and our professional level, which I think is what's most exciting to me. But what I wanted to ask you as we wrap up here is for our audience who wants to potentially follow up, learn more about what you all are doing at BrainBox, what and maybe just follow up on this conversation in general, what's the best way that they can do that?
- [Jean-Simon] Oh, I would say contact us. We have a marvelous website with a lot of content, and we're always very happy to engage a discussion on the topic. So brainboxai.com is I think is the best way to reach out.
- [Ryan] Well, really appreciate you taking the time to be here. You were a fantastic guest. A lot of good insights. Excited to get this out to our audience and hope to talk to you again soon.
- [Jean-Simon] Thank you very much.
Special Guest
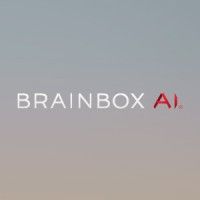
Jean-Simon Venne
- Co-Founder and CTO, BrainBox AI
Hosted By
AI For All
Subscribe to Our Podcast

YouTube

Apple Podcasts

Google Podcasts

Spotify

Amazon Music

Overcast











