In this episode of the AI For All Podcast, Hans-Martin Will, Chief AI Officer at LegalMation, joins Ryan Chacon and Neil Sahota to discuss the impact of AI on traditional industries like legal and insurance. They discuss the role of a Chief AI Officer and its growing demand, the concept of hybrid intelligence, and the potential risks and challenges involved in adopting AI technologies, focusing on transparency, explainability, and legal implications.
About Hans-Martin Will
Hans-Martin Will is a seasoned technologist and product leader with two decades of experience working at the intersection of applied research and product innovation. His expertise primarily centers on the development of data platforms, distributed systems, spatial computing, and the application of AI and ML across various domains, including enterprise business applications, language technology, and life sciences. He has led key initiatives at industry leaders like Amazon, Microsoft, and SAP, as well as product innovation in nimble start-up settings. Hans-Martin holds a PhD in Computer Science from ETH Zurich, Switzerland.
Interested in connecting with Hans-Martin? Reach out on LinkedIn!
About LegalMation
LegalMation leverages the latest artificial intelligence systems (including large language models such as GPT-4) to help law firms drive efficiency with straightforward and easily deployed solutions with a focus on litigation and dispute resolution workflows.
Key Questions and Topics from This Episode:
(00:26) Introduction to Hans-Martin Will and LegalMation
(02:06) What is a Chief AI Officer?
(04:49) AI in the legal and insurance industries
(11:04) Challenges and hesitations in AI adoption
(13:15) Example of AI in legal work
(18:49) AI hallucinations
(22:08) How will AI evolve beyond LLMs?
(25:33) Benefits and drawbacks of AI integration
(27:54) Will there be AI robot lawyers?
(29:09) Learn more and follow up
(02:06) What is a Chief AI Officer?
(04:49) AI in the legal and insurance industries
(11:04) Challenges and hesitations in AI adoption
(13:15) Example of AI in legal work
(18:49) AI hallucinations
(22:08) How will AI evolve beyond LLMs?
(25:33) Benefits and drawbacks of AI integration
(27:54) Will there be AI robot lawyers?
(29:09) Learn more and follow up
Transcript:
- [Ryan] Welcome everybody to another episode of the AI For All Podcast. I'm Ryan Chacon. With me is my co-host, Neil Sahota, AI Advisor to the UN and one of the founders of AI for Good. Neil, how's it going?
- [Neil] Doing all right, Ryan. Just trying to stay dry. We're going through kind of a hellacious set of thunderstorms out here on the West Coast.
- [Ryan] Today's episode, we are going to be talking about how AI will impact more traditional industries like the insurance industry, like the legal industry. And to discuss this, we have Martin Will Chief AI Officer at LegalMation. They are a company that is leveraging AI systems to help corporate legal departments and law firms drive efficiency with very straightforward, easily developed and deployed solutions, specifically focused on litigation and dispute resolution workflows.
Martin, welcome to the podcast.
- [Hans-Martin] Thanks so much for having me here, Ryan.
- [Ryan] So let's kick this off by having you give a quick introduction about yourself, LegalMation, what you all are doing, just kinda your background experience, and then I wanna pull the thread on your role too because I think it's interesting to learn more about what a Chief AI Officer actually does.
- [Hans-Martin] I've been in the language and machine learning space for maybe a good two decades. A lot of that early on in the language technology space. Then almost a decade around life sciences. And then returning back to the language space, particularly with my work at Alexa and also being part of the team that built and launched Amazon Translate, so the first neural machine translation service that went live on AWS.
With that, of course, a company like LegalMation is a great fit. And LegalMation specifically is a company that's been around for a couple of years now. They launched the product around 2018, sometime back, and they're refocusing on bringing AI technologies into the legal space, the insurance space. It's very much focused on specific applications like litigation where we can immediately deploy AI in a meaningful way that directly drives productivity and consistency in our customer organizations.
- [Ryan] Tell me, just regarding your role as Chief AI Officer there, I know you mentioned that you recently joined the company, what is the role predominantly focused on and how does that potentially relate to similar roles in other industries that you may have had exposure to or maybe you have colleagues that are in similar roles, other industries, because I think this is a new role that a lot of organizations may not have, but as the future kind of progresses, it may be more important to be looking into these AI specific roles.
- [Hans-Martin] As a Chief AI Officer, my focus is really on defining the strategy around AI and machine learning technologies. Also driving some form of applied research, implementation, data science, and then also overseeing how these technologies are incorporated and productized with our solution suite.
And that kind of, it's an interesting role because it requires quite a broad set of skills, which is also represented in my team, right? So my immediate team has both data scientists and legal experts plus some data engineering skills, right? Because these are all the different aspects that come together here. I'm also playing a little bit the role of a Chief Data Officer, so I'm also generally widely responsible for how we manage the different data assets that we collect over time, obviously, and how could we generate more value out of those.
- [Ryan] Neil, from your perspective, with the work that you do with a lot of different companies, have you seen this role of Chief AI Officer or just AI roles in particular grow in different industries that you've associated with?
- [Neil] Straightforward answer is yes. I think you're seeing, much like we saw with the CRO, Chief Risks Officer, about eight years ago slowly build up, and it's become a core component for a lot of businesses, I think you're seeing the same thing with the Chief AI Officer role, so it's become a core component of strategy. But I think a lot of people have started realizing, or a lot of companies, I should say, have started realizing that AI is more than just technology. It's not like a traditional IT project and because of the training and the data and the domain expertise that's required, it's a much different mix, and so as a result, it's getting essentially, carve out is not the right word, but I can't think of a better one in terms of functions within a company.
And as I'm sure we'll probably explore in this conversation, not just the power and the value that AI adds to the organization, but some of the risk associated, especially liabilities, are paramount concern that you need that more dedicated focus. So, I think five, if we have this conversation in five years, we probably see that CAIO is pretty much the norm at most companies.
- [Ryan] I'd like to bring this back to the topic and main focus for our conversation today, which is AI's impact on more traditional industries like insurance and the legal space. Can you talk to us about how AI is really impacting those spaces? Because there seems to be a lot of opportunity for automation in those industries.
- [Hans-Martin] No, it's a good question. And I would think about two different categories of work where AI can be deployed. And maybe the first category is really the kind of applications that where consistency is of value to the work. And in fact, that's what we see a lot say in these litigation cases, right? Where if you're an insurance provider, you are a common effector. These cases come in high quantities, right? And you wanna be consistent in how you respond to them. And but that's an also kind of the opportunity to automate and essentially bring in the technology that supports you and streamline these processes, driving up the consistency and overall being more successful in the results you achieve working through these cases. The second category, and we actually see a lot of that more covered in the press right now, particularly since ChatGPT came online, is more where I'm applying AI as a, kind of as a research tool, right? So there's a lot of information I had to process, distill, bring down for a question I have in mind, and the system supports to extract that for me, summarize it for me, often even identify what the relevant information is in the first place. And I would say while there have been a lot of advances being made, there obviously is still challenges in how to get these kind of systems to a level of performance that really matches human performance.
- [Neil] Martin, I think you're touching upon a really important point, right? That the accuracy and, as good as we get today with people doing the work, but there's, there seems to be also a disconnect, right? People are worried, is this really gonna be as good as people do today, but then they're also like, that's actually not acceptable, right? They seem to expect the machine to be perfect. And so if it makes a small mistake, if it's only right 98% of the time, people worry about that 2%. Especially in an industry where risk is paramount and legal and insurance, how do you try and reconcile that attitude?
- [Hans-Martin] It's a good question, and also in the sense that once you introduce technology like ours, you actually get much better insights on say what are the inconsistencies across work you're actually doing in your company right now? But nevertheless, and as you are calling out, what often when you bring these assistive technologies into the workflow of an expert, right? They will pick up on the specific details of specific cases versus what is really happening at the overall level. And I think risk, as you're talking about, is really about looking at, right, an overall portfolio of things that are happening versus every single one of them, and it just seems to be human nature to focus on these individual outliers, so to speak.
I think nevertheless, probably what makes sense, I know what we are trying to do is a, putting humans in the right places in the world of decision making flow, right? So that ultimately there's some form of accountability built into the system and a human is comfortable with the final output, right? The final outcome, even if a machine has been involved in creating that and, say, accelerating putting that together.
And then as part of that, I think it's also, and we talked a lot about explainability in the past. The discussion has been a little bit less vocal right now, I think, but still, I think explainability is actually a key piece here, right? A key ingredient that, the system not only needs to provide an answer, it also needs to help you understand how it got there, right? And by creating this kind of transparency again, it helps overcoming that psychological barrier of can I trust this thing now in front of me, or is it just a complete black box, and I have no way of knowing what I'm doing when I press the submit button, so to speak. So I think that transparency and explainability needs to be part of how we roll out these technologies into the industries, in particular when it's about managing risk, right? So that risk and the decision-making and the trade-offs are transparent to the human still carrying the final accountability.
- [Ryan] Do you feel like that's going to open up the need for different roles within organizations to help with, obviously, when you bring in tools like this, this will allow for certain tasks to be handled and be automated, which requires less need for people, but I imagine that because there is still a level of review and a level of oversight that is needed, there's going to potentially be demand for other types of roles or more of an existing role because of a shift in the way things are being done?
- [Hans-Martin] I would think so. And, I mean, there's a good example. So we recently had a call with a prospective customer. I can't disclose the name here, but one of the conversations in setting up the pilot with them really was them doing an internal assessment of exactly these aspects of any AI technology they're bringing into the organization. To what extent, right, has it been validated. What's, can it be controlled by them? What risks do they expose themselves? So there's, for example, I think a new set of roles really coming around risk management, particularly for intelligent technologies that are being integrated into the workflow. And I could imagine that also the role of a Chief AI Officer in one of these companies would have a close relationship to these risk management activities. So there's really a strategic trade-off of how to set this up and how we think about ensuring that say we meet our compliance requirements and don't put our business at risk ultimately.
- [Ryan] Have you seen certain challenges or hesitations when it comes to the adoption of AI into the legal and insurance space? Like what are the biggest hesitations these companies have? And I guess what are the risks associated with using something that is free out there like ChatGPT versus something that you need to pay for and is maybe more targeted and focused on the, your particular industry or vertical of some kind?
- [Hans-Martin] As I mentioned, I was earlier thinking about these two different categories of technologies that come in. And a lot of what we are doing is selling this first category, which is about consistency and streamlining anx existing workflow. And a lot of the conversations that I think we see internally at our customers is what is the workflow change that comes out of that for them? And suddenly you had say groups of legal experts working rather independently, right? Suddenly, they need to align more closely in the responses, in how the documents are structured. And that often then becomes a primary conversation internally, right? So it's almost more an indirect response to I'm bringing in consistency because I talked about consistency, but now I need to really update my internal way of working around that technology.
On the second one, I think that's gonna be where the risk management piece, right? And the performance capabilities are still a big question mark. Once I go, it might have this technology that supports to support me in my research, and particularly if it's research that goes beyond what a human could even possibly do, right? I think that's part of what we're doing with this technology, right? They have been sometimes given access to so much information that no human individually could digest and understand. So how can they actually see if a specific outcome is even represented in a meaningful way in this body? And so there are definitely, a lot needs to be done to drive this explainability, transparency, some way of allowing the humans to work backwards from an output, from an end suggestion, for example, and at least understand the plausibility of what the machine is really proposing here.
- [Neil] Martin, for our audience, if we walk through an example, since we didn't really talk much about what LegalMation does, maybe we can, Martin, you can share what LegalMation does and walk us through how some of these things factor into the work that you guys actually do.
- [Hans-Martin] We can just take one of our kind of concrete product offerings, right? Say a complaint response workflow, and you'll see as I talk it through, it's really an assistive technology in a sense. So what you do as a user, right, you get this document making certain requests to you from an outside entity, and you need to create a letter that provides the response to all the points raised in that incoming document. And so what our systems do, essentially as a user you would upload that incoming document, we perform first, of course, standard document extraction, but then the next step is a deep semantic analysis, right? What is it that is being talked about in the document and also doing analysis, what kind of concerns based on how you respond as an organization in the past are probably key to framing your response, right?
And then with that, essentially it becomes a workflow where the system looks at previous way of how the responding letter was structured and what was the content, what was the phrasing, the language. And together, right, with this overall classification of the risk profile at the point that we think are important, cause of action in the legal terminology, right, we put together essentially for each of these requests, a ranked list of what may be the most meaningful responses and where were they used in the past and what were other attributes maybe that were relevant in that, right? And then as a user, you can review that, you can edit it, you can override what the system is proposing, and then essentially that becomes additional content that goes back in the system ultimately for future work, but also then essentially the letter is more or less ready to be converted to PDF and sent to a customer if you feel confident in approving that overall document that has been created. So the human is very much closely involved in making the final call. And also we really try to provide, right, the context of why specific, say a specific way of answering here may be preferred to another option, which is still also presented to you on the side as an alternative option.
- [Neil] Good example of hybrid intelligence. We always talk about on the show that there are things that machines are better than people, but there are things that people are better than machines, and it's the complement of the strengths that really drive this and so. Much like we should be treating gen AI, it sounds like your AI system is doing that first pass and providing some options for then people to consider. In normal work, maybe you can consider two or three things where here you might be able to consider 10 different options or do a deeper risk analysis based on the complaint or the responses.
- [Hans-Martin] For me, it's an interesting parallel to what I experienced in the pure language space, localization work maybe two decades ago. Because also starting even in the late 80s, I think IBM first, started promoting the idea of these translation memories, right, where again, you brought in these assistive technologies that would still support the human expert, the expert translator, often domain expert. For example, and the European Commission, right, has, is employing a lot of experts who are both translators but also have said enough about the regulations that it can do meaningful translation work, right? And incrementally, these systems became more capable in how well these suggestions work to the point where in around 2000 we had reached a point where the bodies that had been compiled out of these systems, these corpora, they became actually the training material for the first generation of statistical machine translation systems, like the kind of technology like a Google Translate at the time, right? And on one end, right, it incrementally found its way into the industry, into the workforce, but also at some point we reached a point, an inflection point where suddenly new kinds of systems were possible because we had at that point learned enough about how that domain works. And I could imagine similar things happening here as well, right? Right now we're still at the point where we need to be very assistive, very closely working with the human, and over time, probably the systems will learn more.
- [Ryan] Do you foresee any type of new liabilities coming into this space as the machine and the AI handles more of the workload, even though I know we talked about humans being involved, but just is there anything that you guys are looking out for that people have raised concerns about?
- [Hans-Martin] I think the liability will come in when it, the machine gets to scope in decision making that is larger than what a human can even oversee. I think that's really when we need to be concerned with, what are the control tools we need to build in that, that kind of traceability is still given. Particularly in these cases where it can be rather costly mistakes potentially.
- [Ryan] I know one thing that people talk about a lot when they're using ChatGPT and other types of AI technology and solutions is hallucinations and false facts, things along those lines. How is that influencing things or what have you encountered or how are you going about combating that?
- [Hans-Martin] To answer, I want to go back to what a chat engine and a language model, large language model really is, right, and a large language model at the core is a system that has just been trained on a large body of text, and the training goal was to complete the text, either by adding more words at the end or maybe different variants where you hide some content in the middle and the system needs to essentially guess what were the words that I was hiding and masking out.
So what these systems learn is really the pattern of structure of language. Now, of course, in order to do this successfully, the system is also implicitly learning quite a bit of how we as humans talk about the world, right? And that kind of gives the language model the power to make others that seem like the language model understands what the world is like. But fundamentally, right, this is not what the system does, right? And then in the chat system, what we do on top of that pure language statistic machinery, right, we call it alignment, right? We nudge the model to give us answers in response to an incoming request that we find useful, right?
But now, let's go to this, these scenarios, right? Where somebody's going with open-ended questions or doing legal research against ChatGPT. The engine, all it does is create plausible text in, and that sounds useful in response to a question I put in, right? It doesn't really understand the details of legal work. And so the way to overcome this, and I think that's happening quite a bit actually, is that you say, I shouldn't be trying to use the language model as a knowledge repository but rather really as what it is. It's a, ultimately it's a thing that can work with language and translate language, right? This can be changing the style, right? Doing a summarization, doing an extraction, maybe some classification of the text. And the knowledge that is used to generate the output, particularly in say a field like ours, right, which is so factual, needs to come from elsewhere, right? And then we see systems where it's either knowledge graph, right? So I have actually an explicit representation of the knowledge elsewhere, and the language model is just used to transform that into something that's consumable by a person or the RAG type model, right? I'm saying I'm actually having a different repository of documents. This is the information and the knowledge I wanna work with, I'm just using the language model again as a translation engine, right? To fuse information together and create a consistent output. And ultimately, it's not surprising, right? These transformers that are underlying large language model, they were really built for machine translation systems in the beginning. The Transformer paper was a paper describing how to build a translation engine from I think English to French or something.
- [Neil] Given the state of things, what's really next then? What's the next evolutionary step that's gonna occur here?
- [Hans-Martin] It is probably that we learn how to fuse the language part of these AI capabilities with adjacent pieces. One thing we already saw is, right, even just going from language to multimodal, right, opened a lot of new capabilities, right? Even to the point of understanding incoming information, right? So when I can upload a document, say in a ChatGPT, right, and I can now ask questions, fairly open-ended questions about the document, and it's providing meaningful answers to it, right? That's, I think, is a first step of the evolution. I think the other part will be now going beyond that extraction and generation capability, adding the reasoning part of it and really meaningfully integrating the structured knowledge with the kind of unstructured language and image world.
And then also the kind of loop that you find in a lot of these kind of models of consciousness, right? There are quite a bit, right? If you go into neurosciences, there are actually some models of how we think intelligent organisms work, right? And that's also the part of a control mechanism in the, right, kind of where do goals come from and how do I compare what I'm generating against those goals. And I think once as these three elements get more and more integrated, I think that's really when we see these more independent assistive technologies come to life. And it's essentially what we see even coming out of the big labs, right? When Meta or an organization like Google is talking about where they're going in the research, that's exactly what they're doing, right? They're bringing the goal orientation, the task generation, and the extractive and generative pieces into common systems.
- [Neil] You can start seeing some of that already happening, especially like with the image or even video data now. So there's a lot of upside. You make this leap to this next step, what's the drawback? Or is there one?
- [Hans-Martin] One of the jokes I saw the other day on social media was like, oh, we are building all these intelligent systems, so the machine can focus on the arts and poetry, and humans become the pizza driver that's delivering the food on the way. But there's some truth to it, right? Because I think surprisingly these technologies have been most successful in areas that we consider so close to what humanity and being human is about. The compositional side, the creative side, these, working with these large bodies of knowledge.
But I also think there's another opportunity, right? Because the other opportunity, what humans are really good at is still that connection to the real world, right? And so this may be more shift that we see, right, that, so some of the things that were considered very important, they just become easier because the system can help us more with that. And the focus of us as humans is really more really living in the real world, right? Of course, ultimately that's what this machine is not right. The machine is still this abstract entity in a box.
- [Ryan] I also think it's interesting if you think about the benefits for an organization. So somebody listening to this trying to understand what the value of this is now and into the future for an organization, when you bring an end-to-end platform into your organization, you get to, not only are you getting more access to data, but you're finding new ways to leverage that data, which I think compounds on itself over time. You're able to also help provide better that data to be able to be used to provide better decision making across the organization, especially in this space, we're talking about legal and insurance kind of industries, what the impact those departments have on a wider organization I think is important to think about. And just generally speaking, you get more access to the understanding of what's going on in these departments, and I'd love if you could maybe expand on how you're seeing that as a big driver in adoption for solutions like this as opposed to just using maybe something that's free out there, something that is more of an end-to-end integrated in to the organization and kinda how this compounds over time.
- [Hans-Martin] No, it's, a good point, and I think what we see is that, for example, as companies are deploying our solution, work with us for some time, there's quite a bit of insights you can derive, which we can also serve with an analytics product that we have, right? So that actually over time, for example, you can understand say what are the patterns of cases coming through?
And for example, it may be a vehicle manufacturer. It's actually quite interesting to see what kind of, right, what these complaints that customer, issues customers have are actually about, right? And this may inform either a refinement of the current model I have in the market, or I may use it to really design my next model generation from a very different view on the risk profile that's associated with having this product out there in the streets.
And so I think that's, for me, a good example of, right, how these insights not only help shape work within that legal department in that case, right, but really help inform other parts of the business of course. But legal often is very downstream costly, but the root cause often is much, much else with the organization.
- [Neil] You know, I'm going to ask the crazy question I'm sure a lot of our audience is wondering about and maybe hoping for. Are we looking at a day where it will be an AI robot lawyer that's going to be helping them out?
- [Hans-Martin] I can say I hope not, well I don't think so. At least not for the foreseeable time because I think, right, this notion of accountability, human accountability is still deeply rooted in our legal framework, right? So there's still the need that it is fully human parties against each other ultimately that are standing in front of each other in court. And the robot, I don't think, can be the complete stand in there. But of course, it may, of course, it will of course be the case, right, that more of the preparatory work and the arguments that are being presented are being created with the support of machines.
- [Ryan] Can you imagine that process from the court hearing all the way through the entire case with a human jury and robot lawyers?
- [Neil] AI has learned to be very persuasive, and people seem to have some intrinsic level of trust when it comes to the machine. Well, It's a machine. It's gotta be right.
- [Ryan] We really appreciate you taking the time. For our audience that wants to learn more about what you all have going on, what's the best way to reach out and follow up?
- [Hans-Martin] Probably the best way is you go to our website, legalmation.com. There's a contact form. I think that's the easiest. I do have a Twitter handle, @hmwill.
- [Ryan] We're excited to get this out to our audience. We really appreciate you taking the time. I think this is the first time we've really dove into kind of the legal and insurance side of things when it comes to the role AI is having, all those areas of business and just generally speaking in those industries. So really appreciate it and thanks for being here and hopefully we'll talk again in the future.
- [Hans-Martin] Yeah, thanks so much for having me, Ryan and Neil, was great chatting with you in this conversation.
Special Guest
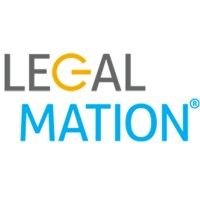
Hans-Martin Will
- Chief AI Officer, LegalMation
Hosted By
AI For All
Subscribe to Our Podcast

YouTube

Apple Podcasts

Google Podcasts

Spotify

Amazon Music

Overcast











