In this episode of the AI For All Podcast, Ben Mones, CEO of Fama, joins Ryan Chacon and Neil Sahota to discuss using AI for hiring and in the workplace. Mones stresses the importance of understanding how AI models reflect human biases. He emphasizes the need for diversity among the teams developing the technology to minimize this bias. Mones also underscores that in this rapidly changing era, businesses need to be flexible and open to new, contrasting viewpoints. Moreover, he predicts the elimination of rote and manual tasks but also the creation of new roles due to technological innovation.
About Ben Mones
Ben Mones is the founder and CEO of Fama, a modern candidate screening solution that makes hiring great people easy. Prior to Fama, Ben held a number of executive roles at a variety of startups in the Bay Area and New York City. Ben is also a mentor on the Go-to-Market Advisory Council of Alchemist Accelerator, which is focused on accelerating the development of seed-stage ventures. Ben has been a guest lecturer at MIT Sloan School of Management, UCLA Anderson School of Management, and USC Marshall School of Business, and has also been featured in CNBC, Fast Company, Los Angeles Times, TechCrunch, and the Wall Street Journal. He holds a Bachelor of Arts from Vanderbilt University and is based in Los Angeles, California.
Interested in connecting with Ben? Reach out on LinkedIn!
About Fama
Fama makes hiring great people easy. Their modern candidate screening solutions use online signals to identify candidate fit, highlighting professional attributes like creativity, innovation, and problem solving while also surfacing costly misconduct such as violence, harassment, and fraud. They help organizations answer that big question: how might a candidate act around coworkers or customers when they join? Founded in 2015, Fama is headquartered in Los Angeles, California. They're backed by some of the world’s leading investors and have raised more than $30M.
Key Questions and Topics from This Episode:
(00:40) Introduction to Ben Mones and Fama
(02:15) Understanding AI adoption in the workplace
(04:50) Challenges and opportunities in AI implementation
(05:11) Navigating the AI landscape: advice for businesses
(07:48) The journey of Fama: from idea to implementation
(09:50) Addressing bias in AI
(10:26) Preparing for AI integration
(27:13) Future of AI in hiring and decision making
(31:38) Embracing change and innovation
(34:51) Learn more and follow up
(02:15) Understanding AI adoption in the workplace
(04:50) Challenges and opportunities in AI implementation
(05:11) Navigating the AI landscape: advice for businesses
(07:48) The journey of Fama: from idea to implementation
(09:50) Addressing bias in AI
(10:26) Preparing for AI integration
(27:13) Future of AI in hiring and decision making
(31:38) Embracing change and innovation
(34:51) Learn more and follow up
Transcript:
- [Ryan] Welcome everybody to another episode of the AI For All Podcast. I'm Ryan Chacon. I'm with my co-host, Neil Sahota, the AI Advisor to the UN and one of the founders of AI for Good. Neil, how are things going from Singapore today?
- [Neil] It's an early morning out here, but this is a great way to start. So we've got a fantastic guest, Ryan. It's going to be a fascinating story.
- [Ryan] Yeah, I'm very excited. For our audience's sake, we are focused today talking about enterprise AI adoption, its role in the workplace and a lot of other topics I think you're gonna find interesting, especially using AI for candidate screening, how that's being used, how that potentially can be used, the pros, the cons, all that kind of good stuff.
And to discuss this, we have Ben Mones, the founder and CEO of Fama Technologies, a company that is very much focused on helping people hire great people more easily. Ben, welcome to the podcast.
- [Ben] Hey, thanks a lot, Ryan. Neil, good to see you again, and yeah, excited to be here. So looking forward to diving in today's conversation.
- [Ryan] Yeah, let's have you give a quick introduction about yourself and the company to our audience. You have a very interesting background. I think it'd be great to touch on that first, give our audience that context as to who you are and what the company does.
- [Ben] Yeah, sure. We've been doing AI since before it was cool. That's the headline. But no, I'm a, I'm Ben, I'm the founder CEO of Fama. We're a talent screening company. So, we try to help companies pretty much answer that big question, how's this person going to act around fellow employees, around customers when they join. Less of are they qualified for a role but as you alluded to much more focused on that quality side of things.
So we help answer that question by plugging companies into the world's richest data stream, who people are online, digital identity, as you exist in text, image, and video online, there's a lot of interesting permutations and extrapolations we can make with that data, whether it's managing downside risk, identifying signs of misconduct, or even looking at professional traits and competencies based on the language people are creating online.
So yeah, that's us. We're a software company out of Southern California but with folks all over the world. And yeah, we've got 3,500 clients, 25 countries. So, we're just getting started out here, but I've been doing it since 2015, so it's a long process.
- [Ryan] That's awesome. Yeah, that's very cool. So let's start off talking about how AI Is being used and adopted in the workplace for enterprise companies, businesses in general, just how are you seeing and how have you seen AI kind of start to become more popular as a tool, a solution that companies are leaning on and adopting into their day to day?
- [Ben] A lot of the initial perspectives and proposed use cases about how AI might've been deployed in the enterprise reflected a lot of how consumers use AI today, right, that drive a lot of automated decision making, routing, et cetera, right? And I think within the enterprise, there are definitely applications where AI on a wind turbine to predict failure before it occurs, right, and do a repair and overhaul, there absolutely are a lot of opportunities for that automated processing, automated signaling, right? But I think as you saw companies start to use it in the world of talent screening, talent enrollment, talent attraction, there were a lot of perils that came up, right? Whether it was like video interviewing, candidate scoring, right? How do you justify that somebody is an 84 on whatever scale a company came up with, right, compared to an 81. What if that 81 has more work experience, right? How do you determine explainability in the models? Have those models been audited? Is there a finger on the scale during the auditing process, right? And so I think a lot of the challenges where we've almost seen a pullback from automated processing overall where companies are now starting to try to find a short circuit on how they can get to human conclusions faster, right?
How can a leader, I know we'll talk about this a little bit later, but how can a leader inside of a company assess the implications and meaning behind what an AI solution and our tool is going to provide to you and apply their very human expertise, judgment to that insight to make a determination about how that might flow into their organization.
So, I think there was this kind of wave of excitement in my world, which is like HR tech, essentially, on we can just get a score for everybody and then we'll red all these other people, yellow these ones, and green everybody through. But, I think the reality in 2024 is actually quite different where that sort of AI might have a home in certain parts of the enterprise, but when it comes to the world of HR talent acquisition, we need to get to a sort of step prior and bring people to the precipice of action rather than tell them what to do. I think that's my, what I've seen in the past couple of years.
- [Neil] I think that's great advice or a great perspective, I should say, Ben. I think what we're seeing from a lot of folks is that everyone knows they should be using AI, particularly for their operations, they just struggle to figure out where those opportunities actually are. And obviously it's a different form of computing, and so they're not familiar with the capabilities. What's your advice to all those people out there? How do they figure out what to do with AI?
- [Ben] I think there's a lot of budget being thrown around and a lot of like top down signaling. I think strategic partnerships or acquisitions for some of the companies that carry the stock market here in the States, you know you can increase market share just by buying an AI company, right? Your total market cap or partnering with one down the line. But I think if you are in a business that is thinking about AI, ultimately that sort of partnership is going to have to generate some sort of real return and in the, zero interest rate days are over, right? And so big companies I think are starting to say any partnership, any innovation, any investment that we make in an AI partnership is going to have a material kind of productivity, call it profitability, free cash flow generation within a business to make sense, I think, in the kind of credit and currency environment that we're in right now. So, I would say for companies that are considering it, we have to look at where are the biggest problem sets within our organization.
What are the things that we thought potentially were unsolvable, the areas that, it's not how do I get a score or how do I reach an automated conclusion about something? How do I outright replace the job that I'm doing? But without a doubt, there are everything from call centers, anything related to text, anything related to audio, right? Customer service centers, right? We've experimented with some of this technology ourselves. So, I would say that's my kind of high level answer. But when you get down to the brass tax of it, I would say set a time horizon and an outcome that you have expected on this experimentation and be real about it because you'll partner with an AI startup or something, they'll be like, oh, you're a first hundred customer, like it's in beta, this is how it's going to work, and then you get maybe 30 or 40 percent of what was promised to you. I think you have to be real about not betting on the come and just expecting very clear outputs from whatever tool or technology that you partner with and. Not to sound too negative or doubtful about it because there's a lot of great technology out there, but I think there are also big promises being thrown around by some vendors, and we need to be real about what value a business is receiving that generates free cash flow or profitability for all stakeholders involved with the company.
- [Neil] Makes a lot of sense. It sounds like you've experienced some growing pains yourself. What brought you down your AI journey and how did it get started?
- [Ben] Yeah, when I, we started Fama, again, we're helping look at digital identity and bringing who we are online and extrapolating insights from that digital identity into material insights we can provide to hiring teams, right? To get a sense of somebody is acting hateful, misogynistic online, right, you're going to look at that as well, is this person going to act that way when they join my company, right, around fellow people who work with me, right? And they're gonna want to leave because of that. Is it going to drive customers away instead of towards us? So when we started the company, we were like Mechanical Turk, using people. We were like that paper wall with a guy on a bicycle behind it, like keeping the lights on essentially and claiming AI on natural language processing and image recognition and what got us down that path when we built our core solution, right, because we don't want to have an army of people reading through a person's online presence, right, doing control F, boolean search strings. It just doesn't invite scale, and there are companies that have gone that path and never really reached that point of operating leverage or strategic growth overall.
And so we got to a point where we were actually able to use the data that we labeled internally to train our algorithms and use person in the loop learning, for example, teacher student models, to be able to get to a I'll just call it gross profit and quality perspective. If you look at speed, quality, cost, ultimately is some of the three vectors you pay attention to, that was really where we began, but you have to start with human quality in our experience of data labeling. That is, we throw the term golden dataset I think around pretty loosely these days, but making sure that golden dataset actually is going to reflect the quality that you want to train a machine on.
And of course, looking at who are the people who are building the tech. How do we reduce bias? Humans are biased, of course, so I think it's antithetical to assume that AI just won't be biased because we as humans have had ways for thousands of years to try to tackle and minimize our own bias, and what we're dealing with now is trying to get machines to do it in a fraction of the time that humans have had over the past couple thousand years to limit our own bias and cognitive bias in a wide range of ways. Making sure you're building AI the right way, but that your data set that you start training on is actually legit and not just something that you make up or buy off the shelf or scrape from some website.
- [Ryan] How can companies that you, I guess from your experience working with companies, how can companies approach being prepared to use AI tools, AI software and solutions that are out there? I think we've done a good job highlighting some of the things to really be thinking about, but if I'm a company listening to this, and I find interest in bringing AI into this part of my organization, is the HR side of things, for instance, how can a company prepare themselves to adopt in the best way possible to lead to the higher chance success when that solution gets implemented inside?
Do they have to have certain data to make this more success, likely to be successful? Or is it something that almost any organization can find success with bringing a solution like this in. I'm sure it varies, obviously, industry to industry, company to company, things like that. But just out of curiosity, what could a company looking to bring this, bring a solution like this into their business understand that they need to do in order to prepare themselves and give them the best chance to success as early on as possible?
- [Ben] Yeah, it's a great question and something that, it's funny, we were just talking about this the other week with our, our tech and our customer success team, but we are starting to see, and this is particularly for large global organizations with what I'll call highly professionalized procurement and purchasing teams that historically, I'd say, five years ago, we started getting questions about infosec, SOC 2 compliance. It became a whole industry, right? Companies like Drata now that, Open Raven that do a great job in this kind of world of data security, infosec, right? Making sure you know where your data is. And because of vulnerabilities within tech stacks and everything from ransoms that were demanded of colonial pipeline or these other big businesses that are out there, I think companies started to identify a strategic risk in how they think about buying technology from a data security and overall I would just say like information security perspective. But what we're starting to see now are these professional procurement teams that have AI specialists that you tell them your model is 99 percent accurate, and they're going to be like what? What was that? Can you, can we talk about that one more time? And I think starting to get to, in the same way that we validate supply chain risk, information and security risk, especially large companies that are out there, we're going to need to start seeing companies apply that same level of AI vetting and granularity to the companies that they're supporting and the companies that they're working with.
And one of our big clients asked to see the F1 scores to look at the precision of our models, asked for AI roadmaps, asked for source technology, where's the data coming from, right? Is this a copyright infringement? There's a lot of questions that we're starting to get asked now that we weren't asked literally 24 months ago. This is like a very new development I think because, unfortunately, I think a lot of businesses got fooled by something bright and shiny that wasn't what it says it was. Anyone can, the lowering of the bar, the access to this technology, I will stand behind forever. There is nothing better than people getting access to fast internet and ease of access to some of these LLMs and developing new tech, new strategies, whatever it is, I think it's fantastic, but that lowering of that barrier has also clouded the market, I think, with a wide range of tech providers that businesses need to get down to, okay, what are we buying and what are we actually signing up for here if, as often in our world of AI software, there's a high price tag associated with it.
- [Neil] I think that makes a lot of sense. But to even do that kind of vetting, there's probably a lot of our audience going like how do I actually even do that? If I'm not familiar with AI, the capability, how do I even get the right people to ask the right questions?
- [Ben] Yeah, I don't have the, I don't have the answer to that one, I think. The challenge is understanding what your bet is. I think some people, some companies are going all in on AI right now and putting a lot of their, I'll call it, maybe not near term but their horizon strategies on artificial intelligence and the promises for future success. But I think finding the people, the technology to do that auditing experts, Neil, like yourself, right? There are people that have been doing this for their whole careers, right? That understand that you can go to an AI boot camp, and you could learn about AI and then we have plenty of our business folks, we put them through a and sponsor like a program through MIT for business people to get more familiar with the use of AI.
And of course, there's a wide range of engineering bootcamps you can go through, but just like taking an art class doesn't mean you're going to be Monet or something like that. And so it's still a very fine craft, and I think we'll start to see more people learn that craft over time, but for now, there is a scarcity of actual, I would say, knowledge around this topic, which can be a little scary.
- [Neil] Is that a two edged sword? Because I know we're talking at the beginning of the funnel. We're seeing more and more companies where their employees are using Gen AI, like something like ChatGPT or Perplexity. I know there's a lot of concern there, and I see companies seem to take an extreme step that either said, okay, we trust our employees, and hopefully they're doing great things and not using confidential information, or if they just ban the whole thing entirely. There's no middle ground. What's your approach or what do you suggest to people?
- [Ben] Everybody at Fama has a GPT-4 license that we pay for. We pick up the cost. We're a smaller organization, we're 50 people, so we're, we can provide that sort of guidance and kind of command and control about how to use it, how not to use it and feel pretty confident in the use of the solution itself.
I think, as in many situations, trying to clamp down on innovation and to limit the overall aperture of what people have access to, just like teenagers trying to get past their curfew, people are going to find a way eventually to get around that with the promise of innovation being as strong as it is. And the Gen AI is stunning. We see implications on a daily basis that are new, whether it's within how our teams work and working more efficiently. The folks that are on Windows or Microsoft in our company are looking at ways they can leverage Copilot on a more regular basis in their day to day pretty recently.
And the signs are promising. But from my perspective as a CEO and founder, I've been doing the company now for nine years, almost a decade into it, my personal opinion is that whatever helps our team innovate, feel more confident in the work that they are doing, and pushing their own potential and what they think they can do, I think it's all good, honestly. So, I'm on the let it ride. I'm not concerned. I think any companies that clamped down on innovation is saying, hey, we don't want to give people a high speed internet. We want them, because who knows what they could do with it. So, I think that sounds crazy now, but I'm sure someone could have said that when everyone was like ripping stuff off LimeWire and Napster and Kazaa back when I was, when I was younger.
- [Neil] You're not far off. I always like to tell the story about the printing press. When it first came out, people actually wanted to not just stop it, they wanted to destroy the machines because they believed it would actually destroy human knowledge. We look back and laugh, right, because that's the opposite of the fact that made it accessible to everybody. So, I'd be curious, if you're a company, and you're concerned, are they turning to HR tech to help them with policy or are they looking for consultants, experts? What do they do?
- [Ben] I think there's a wide range of like influencers, consultants, advisors, I think Twitter, X is now a big place where there's a lot of this like information exchange. Reddit, again, if you know where to look is one of the best websites for this sort of stuff, of real users out in the field who are talking about the way that they're pushing their own innovation.
But yeah, I think companies, we came up with a policy at Fama through HR, And we actually had it in partnership with our data science team of asking kind of data science to help us get our best. You're building the technology, right? So how should we measure in the future? And I don't think we have come up with a templated approach that everyone should use, but we have found a lot of flow through in terms of increases in productivity based on the policies. And at a company like ours where we do deal with personal data, we have to be really explicit about what you can and can't use the technology for.
It's, I think, being explicit and trusting your team to, like you, you have to trust people in this scenario. If you don't have trust, then I don't think this gets off the ground.
- [Ryan] You mentioned something earlier I wanted to go back to talking about biases. And I think obviously anytime you're talking about AI, data always, and especially stuff that deals with where humans are really getting involved, whether it's the data that's being pulled or just the interacting with the data, there's always that concern. So how do you, in the space that you're in, obviously the ramifications for the results and the impact and what it's helping inform businesses around is pretty important for the hiring a company does, the people that are looking to get hired. So how do you deal with something that or deal with biases? How do you approach it? How can people feel more secure that this is a top of mind thing when I know it's a pretty popular question that comes up anytime AI is talked about being brought into a business to help them make better decisions or become more efficient or anything like that.
- [Ben] And it is a, this is a bit meta, but it is a human bias too to forget just how biased we as people are, right? And how long we've had to deal with those biases in a wide range of ways. And I think that the reason it comes up, Ryan, as much as it does, one, because it should, because it's important, right? It has to come up in these conversations because like you just said, people's jobs are on the line, right? Their dream job is on the line, in some case, right? So we got to get this stuff right. I think the real answer to your question, there are obviously the, the audits, independent audits, right?
New York has come out with legislation as recent as just a couple of months ago on how AI tools can be used in hiring. We're seeing that with the AI Act as well over in the EU right now. So legislation is beginning to drive behavior in the private sector as it relates to this very problem.
And that includes, I think, ensuring that the bias aren't, the models aren't biased, testing those models against data sets that are diverse, right? And being able to also make sure that the tuning, because there are ways that the learned listener knows that you can tune algorithmic models to look non biased based on a data set that you run it on by essentially putting your thumb on the scale and over tuning, right? So making sure that there isn't any over tuning of those models itself, and I think the EEOC, the enforcement division there is quite progressive and thoughtful and very future oriented about how to manage this sort of thing. I think they paid attention to it before this got to the fever pitch that it's at now.
But outside of those highly technical, here's how you test a model for bias, right, I think you have to look at who's building the technology, right? Who are the leaders of the teams? How are they setting vision, defining roadmap? And within those teams, does the, frankly, the ethnic, gender makeup of those teams reflect the communities that this technology is serving, right? Because even again, you will never be perfect of removing bias from a solution. There are thresholds that, in some ways policy and the government has defined of exactly what is biased and what isn't, but I would argue every model has some degree of bias in it based on who was building it, right?
And so I think the question is how do you minimize that what I'll just call, sometimes we talk about the sort of a sales chain of command, right? That if somebody tells you that a model is audited, and they have a financial stake in answering that question, that could be deemed as maybe not going to hold up. And that's a lot of what the EEO is saying where these independent audit firms have now come out to audit a lot of these algorithmic models, especially those that are used for hiring. But I would also almost argue that beyond just the sales chain of command, the development chain of command too, if you think about who's actually building this technology from board to CTO to AI eng to data scientist to the front end developer themselves, there are meaningful implications here that I think companies need to take a hard look at. And the reality is too that the industry that we're in, which is technology is one that isn't very diverse, right? So it's a very challenging problem. I think to solve that requires a super thoughtful approach.
- [Neil] You're touching upon I think an interesting challenge Ben because, you know this, I forgot who said it, but all data is biased. The, I know everyone says can we just strip out the bias, it's just not possible. Especially the implicit bias.
So, I guess the question becomes is how do we figure out what is good enough? Because I know a lot of people are concerned about AI replicating our bias, maybe taking things to the extreme, but at the end of the day, we as humans are already biased. Why does that seem to be less of a concern than with AI?
- [Ben] I think unfortunately, we as humans assume that we're perfect. Plain and simple. I think that is a natural thing that even the most modest among us assume that we are operating at a capacity of any algorithm coming into my world is going to make me more biased and not less when in fact most people are very biased.
The simple fact is we've just had, like I said before, thousands of years to mitigate that bias in how we interact with each other. The social dimensions we set up, the institutions that we've built, the, even way we think about like how cities are built, right? So much of this is designed to limit our bias and daily interactions that we have.
So I think there is a first acknowledgement and recognition that kind of like you said, we are biased, right, as humans, right? And every model is going to have bias. How we define the threshold, I think that the EU is a great, a great example for the rest of the world, and I would argue in some cases they're really defining this kind of modern Magna Carta about how data and algorithms can be used because it has to be a collective agreement between the private, public sector, and citizens. Plain and simple. So, I think that's the challenge, is figuring out where that line lies. But the New York, the act out of New York is a big step in the United States, at least in that direction, but I think the AI Act has a lot of contours that we'll start to see replicated throughout the world over the coming 5 to 10 years.
- [Ryan] I guess one of the questions I wanted to ask kind of as we get to wrapping up here is as companies start to bring in more technologies like this, they will have access to more information to help make better decisions, and something you mentioned early on in this conversation was around the ability to, was that automated decision making, how that differs from just having access to data and being able to then make decisions off of it. How, when you talk about that, what does it exactly mean about regarding companies adopting automated decision making for the business and kind of how can companies be set up to succeed there and just be able to handle that when it comes to bringing new insights via AI into their business?
- [Ben] Automated decision making to, to me, and I'm sure the Google definition of this will be a lot more accurate, spot on, but automated decision making to me means reaching a conclusion, in a lot of ways. And a conclusion that is defined within a framework that we understand as people that takes us all the way home, meaning stop, go, red, green, yes, no. These very fork in the road, clearly non gradient decisions about someone or something. That's more when I say automated decision making, what I refer to. And you see a lot of companies that have offered that. I'm not going to name names on this podcast and knock anybody, but there are a bunch of big companies that are in active lawsuits right now, an active litigation about scoring certain individuals based on what they look like lower than their white counterparts, just to put it quite simply. So, I think when you step back from providing that data, providing that insight, we need to go further up the decision making chain for people and how trained professionals actually make decisions, right?
What do I want to know? What do I know about in my world. Hiring. Bringing a person into my organization. Okay, I know that I want somebody with these leadership skill sets. I want someone who's a good communicator, right? Because I know from my experience as a human running this team that communication and honesty are key vectors that are important to building a great team within my organization.
Now, applying that sort of insight that you, and you get this from a lot of assessment tools that are out there. There are a lot of companies that provide that sort of information today, but I think using AI to accelerate that process, to use data, to do it in a way that's frictionless, that's where the kind of unlock is. So, it's almost taking the same technology that we've been using for a long period of time, but shortening how we get to that same conclusion and that same answer, but not saying hire John, not Jane. It's more here are the things that could make a great hire for John. Here are the things that could make a great hire for Jane that you already know. And, but here's how I got that information without you ever having to talk to somebody. Like that, that's where I think the kind of future of this technology landscape lies.
- [Ryan] Yeah, and that was going to be my next question was about the, where does the future of this go? Where, how do people, how should we people be thinking about this evolving over time?
If you just look at how decisions have been made during the hiring process 10 years ago, five years ago, and now what we're talking about in this conversation today, it's dramatically changed. Not just the access to information, but how we evaluate the information, the data, the information sources are very different. Social media wasn't taken into account 10 years ago, probably, to to the extent that it is now. And so how do you see this kind of growing forward? What do you, where do you see the most demand in the conversations you're having for the next iteration of this type of AI solution to help companies better make hiring decisions, better just make decisions in general in the workplace so that they can build the culture they want, that they can have the organization on a path towards success and hopefully limit the amount of not say bad hires, but hires that just don't match what they need. And that's, I know, usually one of the biggest contributors, or I guess things that slow down an organization at times is hiring. I think it's one of the most important things a company can do well is hire well, and this is obviously contributing to that. So, where does this kind of go from here? What, how should people be thinking about in the future?
- [Ben] Yeah, I think how should people be thinking about it? The piece of advice I always provide to folks when asked this question is be willing to be flexible of mind. Be open to a contrarian point of view. Be open to that kind of disagreement, that adversity because we are living through a period of, when the historians write about this period of time, a rapid evolution and change in how technology is affecting our lives day in and day out and behaviors that, you know, we might have looked at even five years ago, everybody looking down at their phone or being on a computer or online or in a Vision Pro headset or whatever it might be, we are living our lives increasingly in these digital spaces and these digital environments, I think a lot of companies are making big bets on that. So, I think the first piece is to recognize that this is a period of rapid change and that you as a business person can position yourself for a trajectory change by being open to that, acknowledging that, and embracing that. So that I think is the first piece is that like you just said this ain't your grandpa's hiring process anymore, you know. We're living in a new time. It's a new period.
And I would say, you know, where it evolves, where it changes, I think you will continue to see rote and manual tasks be eliminated. I think there are people in companies who are doing jobs today that will not have those jobs anymore because of automation on information collection, information segmentation, and information organization.
So, I think a lot of those jobs will disappear over the coming years. And I think at the same time, many new jobs, new roles will be created based on this innovation, and the people that are able to discern the uniquely human as more of these tasks, the ability to get qualified for becoming a welder, becoming a software engineer, becoming a graphic designer, right, all of these things are becoming easier and easier to be qualified, but how do we ascertain that uniquely human piece of who we are that makes us successful? How can we find technology that helps us understand who we are as humans. I think that really is the future that we're up against, but I would just say, the greatest innovations happen when people are open to two opposing points of view at the same time.
- [Neil] We've touched upon a lot of different areas. How do we figure out where the opportunities are, how AI has been integrated into HR tech or maybe talent tech. Some of the biases, but the underlying themes here is still people, that we need people to actually make all of these things happen, and that's why I think throughout the entire conversation, it's again, a great example of why we're moving towards hybrid intelligence, that we're really complementing our human capabilities with machine abilities.
And I think, maybe it's a bit ironic that even in the talent world and what HR does, human resources, it's a powerful tool for, yeah, it's a powerful tool for hybrid intelligence there. Ben, we got to thank you. If people want to learn more about you, what Fama is doing, what's the best way for them to stay in touch?
- [Ben] Yeah, I would say, follow me on LinkedIn. Last name is M O N E S, first name Ben. But also come to our website, fama.io. We've got a lot of innovations, not just coming out from our company, but we've also written this great ebook on automated processing, how compliance or a compliance oriented approach to using this sort of technology with the AI Act, the GDPR, especially if you're abroad, can help you advance your understanding.
So a lot of knowledge on our site. But I'm just grateful for the opportunity to chat with you guys and join you on the podcast today. So thanks a lot for having me.
Special Guest
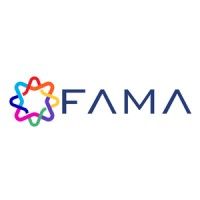
Ben Mones
- Founder & CEO, Fama
Hosted By
AI For All
Subscribe to Our Podcast

YouTube

Apple Podcasts

Google Podcasts

Spotify

Amazon Music

Overcast











