In this episode of the AI For All Podcast, Stan Schneider, CEO of Real-Time Innovations, joins Ryan Chacon to discuss the importance of data in AI. Stan shares how his company works with real-world systems, touching on the complex challenges of software-defined systems and the transition to data-centric design. He also details a range of innovative projects, from autonomous vehicles to medical systems and renewable energy. In addition, Stan emphasizes the significance of sensor fusion in increasing data quality.
About Stan Schneider
Stan is the CEO of Real-Time Innovations (RTI), the world’s largest software framework provider for autonomous systems. RTI software runs over 2,000 designs including the largest power plants in North America, the Canadian Air Traffic Control system, NASA's launch control system, nearly all Navy ships, GE HealthCare's hospital device networks, Siemens wind turbine farms, trains and metro control systems, and over 250 autonomous vehicle designs. Stan holds a Ph.D. from Stanford in Electrical Engineering and Computer Science with a focus on autonomous systems.
Interested in connecting with Stan? Reach out on LinkedIn!
About RTI
Real-Time Innovations (RTI) is the largest software framework company for autonomous systems. RTI Connext® is the world's leading architecture for developing intelligent distributed systems. Uniquely, Connext shares data directly, connecting AI algorithms to real-time networks of devices to build autonomous systems.
Key Questions and Topics from This Episode:
(00:25) Introduction to Stan Schneider and RTI
(02:18) What is a software framework provider?
(04:38) Importance of data in AI
(08:34) Challenges in data collection and quality
(12:28) Transition to software-defined systems
(13:58) Evaluating data quality in real world systems
(16:00) Challenges and benefits of software-defined systems
(18:43) Industries benefiting from software-defined approach
(19:36) Learning from autonomous vehicles
(20:49) Learn more and follow up
(02:18) What is a software framework provider?
(04:38) Importance of data in AI
(08:34) Challenges in data collection and quality
(12:28) Transition to software-defined systems
(13:58) Evaluating data quality in real world systems
(16:00) Challenges and benefits of software-defined systems
(18:43) Industries benefiting from software-defined approach
(19:36) Learning from autonomous vehicles
(20:49) Learn more and follow up
Transcript:
- [Ryan] Welcome everybody to another episode of the AI For All Podcast. I'm your host, Ryan Chacon. My co-host Neil and our producer Nikolai are out today, so it'll just be me with our very exciting, fantastic guest who I will introduce here in a second. We are going to focus today's conversation on the importance of data in AI, how AI gets data, or how you can get data for AI and many other very exciting topics that we have planned.
So on today's episode, we have Stan Schneider, the CEO of Real Time Innovations. They are a software framework company for autonomous systems. Stan, welcome to the podcast.
- [Stan] Thank you. Hi, great to be here.
- [Ryan] Great to have you. So, the first thing I wanted to ask you to do is if you wouldn't mind giving a quick introduction about yourself and the company to our audience.
- [Stan] I'm Stan Schneider. I'm the CEO of a company called Real Time Innovations. We call it RTI. We are on a mission to run a smarter world. We really are not an AI company so much as a framework, the software architecture that allows AIs and advanced algorithms to connect and run real world systems.
So, we're trying to really run a smarter world. So, we do lots of things, over to where we started out in autonomous vehicles. We're in over 250 autonomous vehicle designs, ranging from flying things, underwater things, forklifts, construction vehicles, mining vehicles, ground vehicles, and lately, passenger cars on roads, but that's a relatively, we've got lots of experience with that.
We do medical systems, patient monitoring, imaging systems, medical robots, over 400 different defense systems and renewable energy, especially hydropower and wind power. I just figured out we're running about 12 percent of the renewable energy in the US runs through our software somewhere.
We've done some big things you may have heard of, like the Canadian air traffic control system and NASA's launch control system, but most of our programs that we work with, we've got about 2,000 of them are smart, big things in the real world, like medical robotics or autonomous vehicles.
- [Ryan] Sounds like a lot of, you're collecting a lot of data, that means, from all these different systems and solutions. If I'm listening to this, and I am not a technical person and just trying to understand in kind of layman terms what it means to be a software framework provider, what, how would you describe the kind of role you all play in the industry for somebody that might be new to this type of discussion?
- [Stan] Yeah. So you actually set me up without knowing it, you used the word collect. In the, cloud world, so intelligence is always about data, right? You've got to get data, you got to get it together, you got to analyze it, you got to figure out what to do. Cloud based AIs have days, months, or years to model what they're trying to model. So, most AIs today are used basically to figure out what ads to throw at Ryan. And everything you do online, it can tune its model to figure out what best to show you. In the real world, data isn't static stuff you can collect. That's why I don't like the word collect. It's real time information that's old very quickly. It doesn't matter where the pedestrian was two hours ago. It matters a lot where it was two milliseconds ago, where it's going to be in the next two milliseconds. And what we are basically providing in this world, cars, planes, hospitals, power systems only have milliseconds, is the ability to get the data they need to the right place at the right time, to the algorithms and back to the actuators, which means motors, things that change the world, fast enough to make it work and reliable enough to break. Intelligence is about data, autonomy, intelligence in the role of world is really about data flow. We still need the data. We just need it way faster and way more reliably than you need anywhere else.
Our job, what the framework does Is, knows where the data is, which is mostly coming from sensors, radars, lidars, video cameras, whatever, getting that sensor data to the right algorithms to figure out what's going on and get the result of that algorithm out to the motor, controller, steering or braking system, whatever, to make that work. And these systems can get large. We have systems, hundreds of thousands of devices in them and trying to figure out which data goes to the right place at the right time, that's what we do.
- [Ryan] That clears things up. I think it's very helpful. We've talked about on the podcast before why data is so important in AI, but I think I'd love for you to talk to our audience about that same kind of prompt of the importance of data for AI to even be something that can be utilized and used to its full potential because you said you're getting data from these sensors, from these connected devices in some capacity depending on what it is out there that's collecting from the physical world. And then that's being fed in to the system to be able to be analyzed and have decisions made off of it. Is there more to it than that as to why the importance, why data is so important to driving AI forward?
- [Stan] Yeah, there's a lot more to it than that because we work with real world systems and real world data is typically lots of different measurements of the same thing from different perspectives. One of the most important issues, for instance, is sensor fusion, where you have three or four different sensors all looking at the same scene or maybe different instruments. A great example is a, we have a hospital system, I suppose I can use their name, GE HealthCare is building this very intelligent, distributed, instrumentation system for patient monitoring hospitals. And today if you walk into a hospital, there's all these machines. I think they have about 300 different kinds of machines ranging from oximeters to ECG monitors and respiration monitors, ventilators, people have heard a lot of the names. Today, they're all separate things. They don't talk to each other. They don't know they're in the same room. They don't know they're connected to the same patient. And the only way for somebody, for an intelligence, a human intelligence today to make sense of it is to walk in the room and look at displays and say that one's correlated with that one in this way. And what their system does is uses the framework, which our framework is data centric, I'll talk about that, what that means in a second, but it takes the data from all these different systems and can feed them into an intelligent algorithm that can now make evaluations of what's going on with the patient based on multiple sources of information. It's a lot of information. And of course, the AI, the algorithms have to be aware of what it means if you have a low respiration rate with a high oxygen contact, I'm not a doctor so. Any medical professional listening to this, don't evaluate me based on situations. But, today, even if you're in the ICU, you're probably only going to have a professional caregiver walk into the room every hour or so and look at these devices and an intelligent algorithm can look at it every second, every millisecond. It makes an absolutely huge difference in the ability of technology to help make that a better application.
Take that and expand it by thousands upon thousands of other applications out there. Autonomous cars is the same kind of thing where you now have LIDARs and radars and vision cameras all looking at the same scene and saying that's a pedestrian, that's a fire hydrant, and it's not likely to move, and then you have to have algorithms that figure out where you are in the scene, which is called localization. And also what is likely to be a problem for you, is that car going to be in your path soon.
- [Ryan] So let me ask you, if I'm a company out there who might have some access to data, but understands the importance of being able to collect more data, what are the best ways or what are better ways companies can go about getting data to be able to feed into their systems and their software to provide better insights, make better decisions, all that kind of good stuff, what, how do you think through that if you work with an organization that maybe doesn't have the best data or access to the amount of data needed to really move the needle?
- [Stan] Let me take a power balancing system, for instance. The data you need for that power balancing system is things like ability of plants to produce energy, loading expectations, in the renewable world, wind projections, weather, and sun projections on solar across some large region. The way it used to work is there were a whole bunch of oil fired plants, which are the only things that are big enough to really matter on grid scale. There were 14 of them with operators 24/7 on the phone talking to each other saying, oh, it looks like a hot day in Phoenix. They're going to need more power, but there's not enough wind in our wind turbines over there, and you turn yours up a little bit, and I'll turn my power generation down a little bit. By doing that, they could balance the grid because it's important for the grid to overall have a balance. And back then the hydropower system was just dumping energy out based on how much water they had, but they realized that as you get more and more renewable penetration out there, this human powered thing can't really balance the grid and also the hydropower. So, Grand Coulee is the largest power plant in North America. That's about seven gigawatts. It's also the fastest plant on the grid, so it can take all that power on and off the grid in about 10 minutes, and they change its mission from just generating electricity to balancing what's going on with the other renewables with the wind and solar because wind and solar are clean energy, but they're actually called dirty power because you can't depend on them. They go up and down with the weather. And with that system, they're able to get a lot more renewable penetration. We aren't, yeah, your AI, I realize most of your AI applications are some organization that wants to run the business better on a timeframe that's probably measured in quarters or something. We're a very different company. We're trying to use, take AI a step out of the cloud.
- [Ryan] That provides benefits to the, to organizations, right? Like in their ability to do things better, more effectively in some, depending on the industry and depending on kind of the use case, but I understand what you're saying with as what the difference is.
- [Stan] Very soon you'll see everything, you're hearing in, especially in the automotive industry, software defined. You're hearing it everywhere, software defined vehicles, software defined defense, software defined automation, all sorts of things like that. And really what that means is taking what used to be very much hardware, old school independent systems and connecting them together and making them connect to an intelligence, an AI, so you can have a smart, a smarter system. It definitely makes these systems and the organizations that run them much superior to what they used to be.
- [Ryan] If you're looking at like the important characteristics of a software defined, AI driven world, data flow is a big part of this, but how would you talk about that point as far as the important characteristics to really focus on when we're talking about software defined and then in the world that is much more driven by AI.
- [Stan] There's a fundamental architectural shift going on out there, and it's called data centricity. The whole idea that in the past, most of these kinds of systems were built up from system components. Like we're going to have a database there, we're going to have a router here, we're going to have a network there, we're going to have sensors here. It's all about the things in the system, the physical characteristics. The new design completely turns that upside, and then you figure out what data flows through them. New design turns that completely upside down where now you think about what data each of the algorithms need and which of the systems need, and you design that first and then you figure out what system components you need to deliver that data when you need it. And that is, it's a fundamental change in the way the world works. It's as big of a change as before we had databases in the enterprise world. And it really is all about delivering the data that you need to these intelligences to make fast, good decisions about how it's going to, what it's going to be able to do.
- [Ryan] How do these systems evaluate the quality of data that's coming in? Good data versus bad data, what data do you use to make the right decisions? How does that kind of work into, to all this?
- [Stan] That's a hard problem. Usually the way you figure out quality of, if you have a quality of data problem, the data in the, in these kinds of systems are coming from sensors, sensors have errors. They get blocked out, they get noisy, they get cut off all together. This sort of, one example is the sensor fusion problem I was talking about. If you have three different ways to measure what's going on, it's high, much higher quality than if you just have one. And one of the, one of the early, I'll use a different example. In the defense world, trying to stop an incoming cruise missile from hitting a ship when you, your way of measuring that is with lots of different radar systems, you're going to have multiple views of those incoming things. You can fuse that together and get a higher quality thing, and you can also keep working with one of them were to get lost. And it's the exact same problem to not hit a pedestrian in a car. You now have multiple sensors that can see that pedestrian and if one of them is blocked or for those cameras don't work very well in fog, radar goes through fog pretty nicely, you can use the higher quality sensor as a way to get good data. And there's mathematical ways to do that, Kalman filters and things, but there's also algorithmic ways to make that work better and in a well trained AI system that might be doing lane detection or something like that is really good at making high quality estimations of what the scene really means to the vehicle.
- [Ryan] So let me ask you then when it comes to the challenges of software defined systems, what are some of those big challenges as far as I'm sure there's elements that relate to autonomy, extensibility, other types of elements of all of this, characteristics of all this. What are some of those challenges and even some of the benefits that companies see with software defined systems?
- [Stan] The biggest challenge by far is that there are already systems out there that are not software defined. In the automotive industry, for instance, most, a modern vehicle will probably have hundreds of little boxes called ECUs, electronic control units in the car. Every one of them has a processor in it. Every one of them is doing a very specific function. They work together, but they can't really, can't update them all together, and they can't easily be changed. And even very practical things like wiring them all together, the wiring harness in the car is the third most expensive part of the car and one of the heaviest parts of the car, and you can replace all of that with a few very higher powered processors and some networking technology, and now it's updatable and can be much more intelligent.
At that level, it makes a ton of sense. At the actual implementing and getting into the vehicles level, you've got all these problems with the OEMs, the manufacturers that have their supply chains, and the supply chains are used to delivering the little boxes with very simple interfaces. Software interfacing is much harder than the old hardware, simple hardware interfaces because simple hardware interfaces just send a few bytes back and forth. Now you gotta send an entire image or a world model of what's going on. It's a very different kind of integration problem. RTI is a, we're really a real world software integration expert. That's what we do. And the problem is to actually bring that technology into a different kind of design, unless you're starting from scratch, which is why a lot of the electric vehicles are much more sophisticated in software. It's very difficult to change your entire mindset and your entire supply chain and your entire development philosophy to be around software. The AI algorithms and in that world are things like sensor fusion, planning, collision detection, all those things need all this data that just isn't available in the current world, and there's a huge challenge to get to where you have a software defined system.
- [Ryan] Are there industries that you're seeing take advantage more of a software defined approach than before or industries that you feel like could benefit from this kind of process?
- [Stan] We see transportation, autonomous vehicles, trains, mass transit, quite a bit going on in there, traffic control, like air traffic control, even some drone traffic, like Canadian air traffic control system. The new urban air mobilities, flying cars, we have some customers doing that. In the energy space, it's renewable generation mostly, a little bit of future grid work, medical, robotics, imaging, patient monitoring, defense, cable, radars, avionics, ground vehicles, simulation and training. That's it and then lots of other things. Sports timing, autonomous vehicles, for instance. You realize that the individual cars don't learn anything. That's not how it works. They collect data from many incidents, and they run it up to humans who actually look at these things and create training sets to train the AIs and that updates the neural net parameters, the weights and things and that gets loaded back down into all the vehicles. That's how the training works in this real world system. It's not, it's not like a human driver where every driver has to learn the same mistakes over and over again. Everybody learns from everybody's mistakes. Of course, the problem in the real, in the automotive space is there's a lot of different situations of what's called corner cases out there. It's very difficult to control for all of them.
But I started to say I started my career crashing cars for a living doing biomechanic impact testing at the University of Michigan, and I learned from that a couple things. One, it's very hard to actually predict occupants in a high speed crash. We'll never actually do a really good job of that. And the second thing is a healthy disrespect for the quality of human drivers.
- [Ryan] For our audience out there who wants to learn more about the company itself, what you all are doing, follow up on kind of anything we talked about today or ask questions, what's the best way that they can do that?
- [Stan] Go to our website, rti.com. You can connect with me on LinkedIn. I don't have a nice easy thing, but just look for Stan Schneider RTI on LinkedIn. I do that social media more than anything else.
- [Ryan] Great domain to get.
- [Stan] That's a long story. Actually had seven figure offers for that domain.
- [Ryan] Stan, thank you so much for taking the time. Great conversation. A lot of interesting insights when it comes to just not just what you all are doing, but the value that the access to data is able to provide in these real world systems and solutions for things that are very important, that have the capabilities and what they can do as things get smarter is exciting to think about across all these different industries, and you all working on some very cool projects. So really appreciate you taking the time to come on and talk to our audience about it.
Special Guest
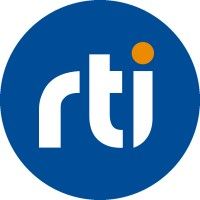
Stan Schneider
- CEO, RTI
Hosted By
AI For All
Subscribe to Our Podcast

YouTube

Apple Podcasts

Google Podcasts

Spotify

Amazon Music

Overcast











