In this episode of the AI For All Podcast, hosts Ryan Chacon and Neil Sahota discuss with Juan Sanchez, CIO of Inteleos, about the impacts of AI on companies and practical tips on deploying AI within organizations. They cover the challenges in integrating AI into decision-making, the importance of having a good data foundation, and the influence of AI hype cycles in organizations. Sánchez also shares his insights on the role of AI in the healthcare sector, the ethics surrounding AI, and his advice for companies looking to incorporate AI into their business.
About Juan Sanchez
Juan Sanchez is the CIO of Inteleos and has over 20 years of experience in the non-profit world. He has worked in design, software engineering, data architecture, and technology management roles. His current focus is on organizational design, helping teams with product development and outcomes-focused strategy.
Interested in connecting with Juan? Reach out on LinkedIn!
About Inteleos
Inteleos is a non-profit certification organization that delivers rigorous assessments and cultivates a global community of professionals dedicated to the highest standards in healthcare and patient safety. They certify over 123,000 professionals across 18 medical certifications in 31 specialties. They represent professionals across 139 countries and those they certify attend to an estimated 1.5 million patients per day across the world.
Key Questions and Topics from This Episode:
(00:25) Introduction to Juan Sanchez and Inteleos
(01:34) What is nuclear imaging?
(02:47) The importance of AI education for companies
(05:29) Incorporating AI into business decision-making
(08:13) The impact of AI on job market and career paths
(17:25) Navigating AI hype cycles in organizations
(23:50) The role of AI in data evaluation and acquisition
(26:59) Evaluating and adopting AI solutions
(33:06) Learn more and follow up
(01:34) What is nuclear imaging?
(02:47) The importance of AI education for companies
(05:29) Incorporating AI into business decision-making
(08:13) The impact of AI on job market and career paths
(17:25) Navigating AI hype cycles in organizations
(23:50) The role of AI in data evaluation and acquisition
(26:59) Evaluating and adopting AI solutions
(33:06) Learn more and follow up
Transcript:
- [Ryan] Welcome everybody to another episode of the AI For All Podcast. I'm Ryan Chacon. With me is my co-host, Neil Sahota, AI Advisor to the UN and one of the founders of AI for Good. Neil, how's it going?
- [Neil] I'm doing all right, Ryan. How about yourself?
- [Ryan] Good, man. Good to have you. So today's episode, we're going to have some really interesting topics to talk about. We're going to be discussing how companies can grow their AI knowledge, and how they can begin thinking about incorporating AI into their decisions. And to discuss this, we have Juan Sanchez, CIO of Inteleos. Inteleos is a nonprofit certification organization. Juan, it's great to have you. Thanks for being on the podcast.
- [Juan] Thanks, Ryan. Thanks, Neil. Super excited to talk about this, especially from this nonprofit angle that I'm a part of.
- [Ryan] Yeah. Why don't you tell us a little bit more about the organization?
- [Juan] Sure. Yeah. So I've been at Inteleos for four and a half years. The mission is fundamentally to certify medical professionals, specifically medical imaging professionals. So when we think about that in layperson kind of terms, it's sonography specifically, right? So anybody that does ultrasound that's our biggest share of customer. And then but we also have certifications in different medical spaces around other imaging types, MRICT, and we do some stuff with cardiologists, so doctors as well get certified in our, now we have an edge, sort of an interesting edge one, which is nuclear imaging and that has a whole different set of regulations around it, which is fascinating to learn about but, but you know ultimately, the mission of the organization is to I think improve health care around the world, and the way we believe that happens is making sure that the people that are out there practicing this stuff know their stuff and can do it safely and accurately.
- [Ryan] Before we jump in, I have to ask you, tell me, tell us about nuclear imaging. This is obviously not connected to our topics here, but I'm just curious when you mentioned nuclear and healthcare.
- [Juan] Yeah, so since I know you're local, Ryan, so if you drive down Rockville Pike, there is a building down there called the Nuclear Regulatory Agency. Big brown building near White Flint Mall. So local reference for anybody in the DC area. So they they essentially regulate all things nuclear in the country including this space. So nuclear imaging, if you've ever had for example a test where they inject you with a nuclear liquid that creates contrast in the imaging equipment, that's the part that's hyper regulated, right? Because they're legitimately injecting you with something radioactive to some degree. I am not a doctor so don't quote me on any of this stuff, but, but fundamentally, and it's wild because it has to be regulated by the NRC.
- [Neil] What happens to the radioactive material after it's injected?
- [Juan] I know nothing.
- [Ryan] Yeah, this is, if you're interested in health care, this is the area to be in. You just drive down Rockville Pike anywhere around this area or even Shady Grove Road where I am right now, it's all massive, like big health care organizations, so it's really interesting to be around here.
But cool. So let's go ahead and jump into kind of some of the topics I know we wanted to cover today. And the first one I want to talk about is really starting at the fundamental level of understanding AI when it comes to an organization. So what, how do or why do companies need to be educating themselves on things like AI and how best can they do that? Because I think that's a, there's a lot of people out there that hear a lot about this, but maybe they're not really sure where to begin or how to really think through what do I, what should I be spending my time educating myself on as as it relates to a company or organization.
- [Juan] Going down first principles thinking for me is important with any of this. I look at it from the point of view of like, all right, what can I solve with this emerging technology? And I say emerging, and I think in actually some pretty big air quotes right here, because it's been around for a long time, right? The hype cycle caught us right now because it's where we are and a lot of tools are coming to market that are very consumer friendly and customer facing. But the fundamentals have been there for a long time, which is how do you use your data to infer new and interesting things? And so inside the organization, I think the guidance I try to give is just use that first principle thinking about what are problems we've had, either new ones or most likely ones that we've had for a long time and can any of the things that we're talking about now or the things that are coming to market now give us a little bit of a benefit or a leg up from, before maybe they weren't insolvable or because we would need more people or we would need data we didn't have or the models themselves didn't exist.
- [Ryan] Neil, from your experience, working with a lot of different organizations through AI for Good and other work that you do, where, what are some thought, things you've come across as far as having to help people and companies educate themselves around AI as it relates to their business?
- [Neil] It often revolves around trying to understand the potential usage and because AI's got a whole new set of tools people have never seen before inside the toolbox, they struggle. Most organizations keep thinking about automation. And so, especially in the nonprofit world, you're looking, okay, what are the off the shelf things I can use to help reduce some of my costs or implement programming. With the, these new tools, there's new things that can actually be done, and that's what I think they actually struggle and that they can actually serve larger populations or constituents with the same amount of resources they already have. Or I've seen now that people are trying to finally understand that some of these AI tools because of the psychology and stuff that the use of marketing and sales, they could actually leverage these tools for improved fundraising.
- [Ryan] So if we go one step further, you've taken time to focus on educating your organization, individuals within the organization, so forth. How do you view or how can smaller companies particularly plan and really think about incorporating AI into their business, into their decision making, things like that now that they've, once they've educated themselves now, it's okay, how do we implement? How do we adopt? How do we start thinking about AI in what is, what we're doing? Which I assume addresses, or it goes back to part of your response earlier around what are the problems that you have, but just curious kind of how that kind of phase is or what advice you have for that phase of kind of the process when it comes to bringing AI into a business.
- [Juan] Recent thinking I've been having around this, and it is somewhat informed by some coursework that I'm doing actively right now. And it goes to the quality of the data you have inside your systems as they sit. I think a lot of people said, oh, AI is here, the CEOs of companies love that because it's a thing to stick on and a way to leap forward, but the data still matters.
And so for me, it's, that's what I'm trying to start to build out is, okay, we are going to use AI, and AI is going to be a thing that is going to allow our customers, is going to allow us like Neil mentioned, you know maybe some efficiencies in our processes, but certainly in I think the quality of delivery of the product we have and so, but we still need to figure out that the data we have is good enough to deploy on any kind of AI tool, right?
Certainly, we want to be mindful of things around obviously about, around bias, and I don't think the goal ever is going to be zero bias. It's just less bias and being informed on that, I think it'd be important. So I think taking the role or framing it around like these tools are going to be assistants to us,they're not going to be the things end all be all solved solutions for everything. And, at least for today, still having the human in the equation considerably. Especially in our medical space, that has to be part of it. Like it can't, we can't go into this with a fervor that says AI replaces all humans. It can't. Just for today, it's not there.
- [Neil] I think that the truth is a lot of organizations have really seen this is that you really haven't automated that many people out of jobs. What's happened is they found that there's a lot of other work that needs to be done, and they're repurposing those people for more value add tasks and stuff. But I've pretty much not seen anyone essentially cut the number of employees because some of this technology. The other thing is a lot of it is not perfect. Anyone that's used gen AI like Claude or ChatGPT or Midjourney, you can see you're getting the draft. It's not the final output. I know that Juan's chuckling because I know he has a lot of experience with that.
- [Juan] Yeah, no, that's right. Neil, and I think there's a point here that I think it's interesting to talk about a little bit, if you guys don't mind, which is, okay, but in a few years from now, as these tools mature and presumably automate some of what we do today as people, I've been thinking about what that's going to do to the pipeline of talent. Almost in a weirdly maybe economic sense. So maybe it's not a, not a perfect comparison, but it's, if we no longer need, let's say, entry level people because the AI is doing the entry level work, then how do you get people to mature into the middle of the company, so to speak, right? And then how do you create senior positions from there if you've completely erased that need for the entry level. I mean, I'm just, you know, I'm just using one particular case, but is that something that you all have heard as well or seen or thought about?
- [Neil] I mean it is from my standpoint. I can answer that in two words, dewey decimal system. That's actually three words I think it's just that the trajectory of the career path is going to change. What we normally think is entry level, there'll be other things that become entry level. So I liken it to the dewey decimal system because I think we're all old enough, maybe not Ryan, but you remember, we had to learn that as kids, and that was a way to find books on the shelves.
Today, you don't really need that. I think they've actually stopped teaching it because it's like you search online with keywords, you find the book, and odds are it's an e book. So you get a copy that way, you don't even have a physical book, you don't need anything. It's the same thing. Like in law, one of the slowest moving industries, sorry to all our legal services fans out there, but some of the work that associates, lawyers are doing around research and reading complaints and filing court documents is all being automated and it's not that what's their path upwards now, it's actually just becoming now they actually focus more on case strategy, some business development or rainmaking. You're working on some of the soft skills, jury selection. These are all still important things, but now the paths are open there that some of the stuff has been automated. The firms could take more work on. It's now okay for them to do it earlier because they actually have more cases available for them to actually hand out to everybody.
- [Ryan] Yeah, and I think there's certain jobs, it's forcing function for people to learn new skills, move up in an organization, or even learn new skills prior going into, I think the educational component ahead of coming into a job is going to have to change and just like it has to where it is today. I feel like that's just natural evolution of a lot of these, a lot of these jobs, a lot of these industries. What was an entry job 10 years ago is not an entry job now, or it might not exist. I think every industry is going to have to battle that kind of on their own, but there'll be a lot of similarities that kind of carry over for sure.
- [Juan] Yeah. There's a good feedback loop there too, right? You mentioned education because that's for sure, I'm not unique in thinking this, but that's definitely a place where AI is going to play big time, both at early education, as well as I can, even in our cases, with professionals that have been in the field for years, constantly having to learn, right? The science, it's the basis of the entire thing. That's for us a huge area about how do we leverage AI to create better educational experiences for them.
- [Ryan] I think it's a really interesting point because if I think back to when I went to school, there was a lot of professors that just over the years had a reputation of teaching the same stuff and just being locked into their tenure and their same textbook year after year, which, but being able to have access to new information, more timely information and be able to teach in a kind of different way, I think is going to benefit. But yeah, it's an interesting thing to think about for sure.
- [Neil] There's an interesting aspect to this in that our learning models are still mainly based from the 19th century. That's actually the way we teach and educate people. We've automated some of those things with VR and AI, but now you're seeing this kind of renaissance in cognitive science where it's like there are actually more effective ways to wire your brain and learn knowledge and develop skills. Couldn't really do before, because technology actually enables that type of learning. And so you're seeing this renaissance and there's this interesting pushback I think Juan will like to hear that people are like, are you trying to fix something that's not broken? And it's like no, we're just trying to find a new way of doing the work. It's not like I'm trying to fix something that's broken, it's trying to find actually a new, better way of doing something. And I think that's where a lot of small businesses, even medium sized, even large businesses struggle with and nonprofits and government agencies in that sometimes we look at these things and we're like, what are we trying to fix here? There's nothing broken, but it's like we use new tools, we can actually do some of these things very differently that would be far more effective.
- [Juan] There's an irony that's not lost on me in that the closer we learn about programming machines to act and behave like our own brains, we start feeding back to ourselves to not teach us like machines, more like humans.
- [Ryan] If we're saying that certain entry level tasks will soon go to the wayside of technology and automation and then the requirement in order to have that new entry level is going to be higher, how do you think that's going to motivate or maybe not motivate people to learn those skills that are required in order to advance their career. Do you think that's going to become more of a challenge for people? Do you feel like people are going to be less motivated to do those kinds of things, or do you feel like there's still going to be plenty of opportunity if, for people who want it?
- [Juan] I'll go with the positive side of that, the more optimistic side, which I think there's going to be plenty of opportunity. And for me the example that kind of hit my brain as you said it was, and I've said this before to friends, the iPhone never came with an instruction manual. The motivation to learn it because of the value that was on, that you were unlocking by using that device in your life was so great that it immediately dissolved any barrier that you were going to put in front of it. Shout out to my mom who uses all the iPhone and Apple things, and she's in her mid eighties.
To me, that's all the proof I need to see. So I think this kind of, these kinds of tools that are going to be coming forward for us and changing the way we work, they're just going to be so valuable to you and to learn them that you're going to just do it either actively or by osmosis almost.
- [Ryan] As certain kind of jobs become less desirable, there'll be more opportunity for those, for people to do those less desirable jobs to grow in their career. So it's more of, just talking about like more manual labor and like physical and hard skill type stuff, like carpentry and things like that, where you really probably not for the near future going to have AI come in and take over those roles, so it created opportunities for people who were not able to advance themselves in those AI generated jobs or the jobs that AI was taking to have opportunity, to new opportunity that maybe people didn't really realize were there. So I'm curious how this is going to really impact people's drive for changing careers, learning new skills, focusing on maybe skills that people thought weren't that important, but maybe now will become because a lot of stuff is being, could potentially be done by AI and things like that.
- [Juan] Yeah, it's funny. I think you're right. I think the trades, for example, are going to benefit from this in not the way that I think, it's the way you're insinuating, which is, I may choose to just opt out of the entire technology world and go be a trades person because that's just a more fulfilling job for me today, right? And I can maybe make the same amount of money as I'm making jockeying AI around. I, in fact, I had an interesting little anecdote here to tell quickly, which was amazing. Friday, I went and got a haircut and, and I sat there, and you usually have your conversation with the person going to cut your hair, what do you want to do? And I said, hang on. So I opened my phone, and I opened up a bunch of Lensa pictures that were all AI generated of myself, different hair, hairstyles. It wasn't about that, right? I actually just Lensa as a fun thing like the rest of us did, so we could put it on our LinkedIn profile, but, and pretend like we're not aging, but so I showed the guy these pictures, I said, look, here's the one that I've been using on all my like internal chat tools and my profile pictures, and he's like that's a good haircut, and he looked at it, he's like this is amazing. He's, has anybody ever walked in here and shown you AI generated pictures? He's like no, but I'm absolutely going to ask people to do that because then we have a conversation about what you want and you can see yourself reflected in the tool. And those pictures are there. He said, but he added this, which is back to the trades idea, you still have to know how to cut hair, right? You're not going to have a robot do your, not yet, do your haircut.
- [Ryan] I don't know if I would trust it.
- [Juan] No, I'm not going to trust that, I mean.
- [Neil] Didn't they have robot barbers in Star Wars?
- [Ryan] Yeah, that's true. I think a lot of time has to go by proving that they will not mess things up for me to trust that. I've been going to the same person since I was like 14 years old, and it's just trust that I know what I'm going to get. Let me ask you this, when we talk about or people hear about things out just through their browsing of social media, their chats with colleagues, these hype cycles are just naturally developed within different organizations and just across society. And when it comes to AI, I think it's been very big hype cycle for the last number of months into the end of last year. How do companies or how can companies navigate that hype and how do these hype cycles really influence things within an organization? Because it's very easy for certain people within an organization maybe chase the latest and hottest thing but in reality, some of these hype cycles do end, and they fizzle out and those things are not necessarily as big as you thought. So how do you evaluate what is worth paying attention to? What is worth learning about to then incorporate and potentially bring into your business?
- [Juan] Yeah, that's a deep question. All right, let me tackle it from first the really pragmatic side, which is when the hype got real, real hype, and my colleagues, the same thing, a lot of calls to, hey, you all CIOs, we need policies and procedures around AI. So that's for like internal to the business, right? That one, I took a pause on and I said, don't think we need anything separate for AI. I think what we need are guidelines, right? So just some sanity checks, which again, I'll go back to, they still, these guidelines that I, and I legitimately wrote them in 15 minutes in a very condensed style and published them and made them freely available to everybody in the nonprofit community. So it was first, back to first principles, check what you're think you're looking at right before you release it to the public. Be careful about sharing that, anything that's intellectual property that you wouldn't share in a public sphere anyway. Assess the cybersecurity risk of the tool, and then move forward with it. Just adapt those, those first principles like you would to anything we use.
I think the new, maybe the nuance or the new thing here is like that trust thing, or don't just assume the thing that the thing spitting back at you, especially if you're using a chat style interface, that those are like the words. And I think the other thing I've added to that in the recent past is the machine isn't lying to you. It is just trying to formulate the best string of words together that it thinks meets its algorithmic need, right, or its optimization. But don't make the mistake of thinking the thing on the other end is a human, that it's actively trying to lie to you. It's not. Right? So that was it for our end, right? Recently we talked about it a little more to see if there was still an opportunity now to do a standalone policy. I'm still of the thinking that you don't because you should have policies already in your organization that speak to a lot of what any of these tools were, would, would expose you to as far as risk.
On the other side, so that was the internal, very like practical, okay, we just got to keep running the business. How do I help people find their way through this? On the other side of it, it's who, who are the platforms, who are the companies that can help us deliver a better product to the customer. So in our case, right, as I mentioned, we are in the assessment business primarily, right? So we write exams. And as I mentioned, one of our key goals is also to reinvent the way those things are done. So naturally for us it's let's look at companies out there that are either in that assessment space or education space that can help us produce a better quality tool that assesses the performance of the people that are coming to us for that assessment and then move from there, right?
I don't think in companies our size, so I know one of the things that we want to talk about is that we're a smaller organization, and we don't have unlimited money, especially as a non profit. So a lot of this has to be done through partnerships. Not the, I don't think the play here for us at least not immediately until costs come down even further, that it's going to be to build our own things. It's absolutely going to partner and leverage what other folks are doing and then also benefit from the wisdom of the collective, working with other partnership organizations that are similar to ours or in the same space as us and trying to figure out coordinating with each other on what we're learning collectively, to help inform each other.
- [Ryan] So you mentioned your role in particular as a CIO. There's lots of obviously CIOs and other organizations that have to evaluate and understand all these different things, different technologies that come around. So how is AI impacting how you've seen CIOs and their role in responding and helping organizations like absorb these technologies that are emerging very quickly. What kind of things are you seeing and what things should be thought about for other CIOs out there that are listening?
- [Juan] Yeah, so guiding principle for me there is take the tool if it makes your life better. So just again super simple. Take the tool if it makes your life better. But only take the tool if it makes your life better, and we're also paying for that tool already because we've seen a lot of, that's been the early thing right now, it's been everybody that we're partnering with from a SaaS platform perspective had to also now with AI, right, extra strength AI in their platform. And so I'm fine with that, but let's make sure that we are at least under a contract or under some sort of legal coverage that if we start using the AI component that they're introducing, that it's not exposing us to unnecessary risk, number one, and then number two, go back to the usability part of it. Is it a toy or is it something that legitimately is adding value to either the way you work on a daily basis or the outputs that you put out as a person that does a particular kind of work or even better, is it improving the outcomes that you're having either as an individual or as a team?
And if the answer to those questions is yes, then keep, then keep doing that. If the answer is, you know, sort of, but wait and see, then wait and see. I think that's been my philosophy so far.
- [Ryan] One thing you mentioned earlier in our conversation that I want to come back to is you talked about, especially for smaller companies, being able to determine and evaluate that they, if they have good data. How does, how do you evaluate, how does an organization evaluate if they have a good data? And if they don't, how do they go about getting that?
- [Juan] That's a great one for some of the work that we're particularly doing right now. All right, so first, first things first is hopefully in your organization, you've made some effort around data governance. And I usually, I hate starting with that because it's such a boring topic in a lot of ways, which is okay, I know where our data is, our databases are properly constructed. I have data definition catalogs published and up to date. None of that stuff is sexy. But it turns out, even though before it seemed like it was just things that you had to do, now it's paying dividends if you have done them or so I think start there if you haven't done them. If you've done them, congratulations because now at least you know where things are. Now, for as long as I've been doing this, and I've been in the tech space for over 20 years, yeah, i've been hearing we have bad data. Our data is no good. We, you know, we need to clean it. We've got duplicates. We got to merge records. We have, okay, so all that foundational data. The bad news is I don't think any of that is going away. However, I think where AI can, it can itself be a tool to unlock itself later for other uses. So what I mean by that is if we think about machine learning as I, and I think fairly, right, you would agree that machine learning is considered a subset or, you know, a part of AI, right? I mean in the conversation, that's a thing. If we can adopt some machine learning knowledge against the data that we do have or that we don't have, and I know in a previous episode you guys interviewed one of the people from Tonic, which does synthetic data, right?
So there's an idea there that can we extrapolate, can we, and really the fancy term, not so fancy, is impute, can we impute data that we're missing in order to increase its quality for us to then do something further with it later? So I think that's a very practical application of sort of the, the foundational AI stuff that isn't the sexy ChatGPT stuff, but still requires modern technology, right, modern machine learning approaches to unlock. So that's where I would guide people to look and say understand what you have through boring governance, ask the business or even better ask your customers what their main problems are. Overlay that with, okay, what do we have and what, where are our gaps and, and then work with some machine learning to fill in those gaps and then eventually deploy that, if you can, deploy that against an AI model that does better prediction for you, for example, so you can tailor your solution to the customer ultimately.
- [Ryan] So the last question I want to ask you before we wrap up here is just around taking everything we talked about today. If you were to give just a couple pieces of advice for people and companies looking to, how to evaluate and adopt AI solutions, how best they can do that to give, increase the likelihood of success, bringing that internally, what would you say to them?
- [Juan] I think there's going to be an emergence of a couple of things. I think one is going to be, so if you're buying an off the shelf solution, and even if it's not, I think there's a question to be asked there about a company, which is can that company audit its algorithm? So I think that's important. If the proposition, the value proposition of some of these models is to, like I said before, reduce bias, for example, just make, help us make better quality decisions that we couldn't make ever before, then I think it behooves us to understand what's inside that black box a little bit. And certainly depending on how we deploy that because we, the last thing we want to do is create a worse situation by accident than what we had before. In fact, I just read an article yesterday, was from a little while ago but how using race data in diagnostic models was creating a really perverse effect in who got treatment and who didn't get treatment specifically around kidney disease, right? So that's the last thing we want, especially again, we're in the medical space. I think that's one. I think the other one, which is an emerging one, for me, just because it's sort of part of the core principles that we want to have, what is, if you're buying again from a third party company and let's say they're using supervised learning for their models, right? Where are they getting the labor for that? Because we've heard horror stories about content moderation on social media platform, right? And the horrors of some, what people have to do and endure to make sure that other people in the world don't see the most heinous stuff in the world, right? I think the same thing applies here, which is not so much from, from you're looking at bad stuff, but more from are you getting paid well for doing labeling work, and do we care about that as a company, right? Do we care about the provenance of where this work comes from? I think that might be, that's more emerging. I don't think there's a lot of people talking about that right now. And I certainly am not going to be utopic and say, oh, just because you can't prove that, you're not going to use the tool. I live in the real world, I think. So I think that's not a thing today, but I hope it becomes a thing further.
So there, so that's the second part. And all of that for me wraps into the ethics. And then finally, it's just, can the tool show us, provably show us an improvement in a particular outcome we're looking for. And I think that just comes back down to wouldn't that be the thing we were asking about everything we've bought for the, for however long we've been doing technology, right?
But I think this is the unique part of this is that, it's just that weird unknown of what is the algorithm doing? And I think pushing the vendors to be able to audit either third party or themselves and show that back to the customer buying that, that, that tool set.
- [Ryan] Yeah. I think it's interesting because if you really pay attention to the space, there's lots of conversations and concerns around bias in models. The less you really understand about the inner workings of any solution or just AI in general, the more hesitation that those kinds of conversations and those headlines can create for people to adopt because the last thing I think people want is for them to bring in a tool that is giving them results that are not what they're looking for, influenced by some level of bias that is not, so basically giving them what they thought they were going to get from a data standpoint or like an output standpoint. So I'm curious to see how that impacts adoption across the board. And then to your, to one of your last points around making sure that the tool or the solution is actually has a kind of tangible output like to be able to evaluate it just like you said we do with basically everything we've adopted in the past, that was something I think it was, was it Marc Andreessen, Andreessen-Horowitz, they put out a whole thing about that technology's great, and it's you know, it's cool to show neat things that your technology can do but if it's not providing a real solution, then what are we doing?
So that is something that we've noticed in, we got our start in the IoT space, and that's what we've seen over the last 6, 7 years, the evolution of showcasing and focusing on technology to now finally showcasing and being focused and understanding the value of a full solution and that being where we are of people wanting that. And that's where we got to get to with the AI side, too. It can't just be showcasing how neat this tool is or what, you know, this technology can do, it's no, let's see how it really works and provides real value to an organization before they invest in it.
- [Juan] You know, I don't want to come off as saying oh, don't take this on and being a Luddite about it. I don't think that's the right play either. So I think there's a way internally for you to strategically deploy this in gates, right? You can certainly adopt AI, but test it as much as you can internally before you start releasing it in, especially to your customer facing world. But and even when you start doing that, just do incremental feature release kind of thinking around it, which is, this is safe enough to try now. Okay, let's put that out into the wild. Now what's, you know, what else can, what's the next horizon out of this feature set that we can leverage. Go through the battery of validation there and then do the same thing and repeat that cycle. And I think that's a pretty reasonable approach to incorporating this stuff versus let's just buy this thing, and we're going to tell the board that all the things do AI. Well, great.
- [Ryan] Yeah. It's interesting to see the use of the term AI when it comes to anything so. Thanks for being on man, this has been great. I really appreciate you taking the time. It's super cool to learn that you're local as well. For our audience who wants to learn more about the organization, what you all are doing, maybe follow up with any questions or thoughts, what's the best way they can do that?
- [Juan] Yeah, so website, inteleos.org. You'll see that there are a collection of other brands under that website. Those just represent our different medical disciplines if you want to call them that, the way we break things out. But inteleos.org is the site and then if you want to get in touch with me, it's just a first name, last name, first name dot last name at inteleos.org, happy to talk there. Or on LinkedIn, but of course I've got a very common name, so that's going to be a hard time. But if you look for me, for Inteleos and my name, you'll find me on LinkedIn. So happy to connect on there too.
- [Ryan] Well Juan, thank you so much for being on here. Sorry that we had some technical difficulties with Neil, but so we'll get that worked out on our end, but really appreciate you being on and excited to potentially have you back in the future to continue this conversation and talk further about what's going on in the space.
- [Juan] Yeah, totally. I would love to. Thanks, Ryan.
Special Guest
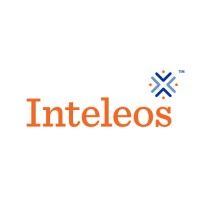
Juan Sanchez
- CIO, Inteleos
Hosted By
AI For All
Subscribe to Our Podcast

YouTube

Apple Podcasts

Google Podcasts

Spotify

Amazon Music

Overcast











