In this episode of the AI For All Podcast, Evan Welbourne, Head of AI and Data at Samsara, joins Ryan Chacon and Neil Sahota to discuss how physical operations in various industries can greatly benefit from AI transformation. Evan highlights highlights unique industrial challenges that AI can solve, such as dealing with large amounts of field data, time-consuming workflows, and risky work environments. He also talks about the concept of connected operations and how enterprises can improve safety, efficiency, and sustainability by digitizing their operations.
About Evan Welbourne
Evan Welbourne leads the AI and Data organizations at Samsara, which includes machine learning, computer vision, data science, and analytics - as well as data engineering and data platform for the company's petabyte-scale IoT data. His current focus is on infrastructure and tools that support and accelerate Samsara's interactions with machine learning, analytics, and intelligent computing systems. Previously, Evan was the Head of ML at Alexa Smart Home and the founder and CEO of AlgoSnap, a company that accelerates the development cycle for edge analytics in the Internet of Things through automated machine learning.
Interested in connecting with Evan? Reach out on LinkedIn!
About Samsara
Samsara is digitizing the world of physical operations. Think of everything from energy utilities and food distribution to transportation and construction - these are the organizations that keep the world running and represent 40% of GDP. Samsara’s cloud platform, IoT sensors, and AI-powered insights make it easy for their customers to act upon massive amounts of data from vehicles, assets, worksites, and heavy equipment. As a result, they can run safer, more efficient, and more sustainable operations.
Key Questions and Topics from This Episode:
(00:39) Introduction to Evan Welbourne and Samsara
(01:07) Understanding physical operations
(02:02) Connected operations
(02:32) Challenges and opportunities in physical operations
(05:23) Impact of AI on frontline workers
(07:10) Future of AI adoption in various industries
(08:30) Leveraging IoT data for AI applications
(09:31) Improving working conditions of frontline workers with AI
(11:30) Addressing concerns around data privacy and security
(13:10) Feedback and learnings from AI implementations
(15:17) Best practices for AI adoption
(17:16) Role of LLMs
(18:43) Getting started with AI in physical operations
(26:23) Evaluating the quality of your data
(28:03) Learn more and follow up
(01:07) Understanding physical operations
(02:02) Connected operations
(02:32) Challenges and opportunities in physical operations
(05:23) Impact of AI on frontline workers
(07:10) Future of AI adoption in various industries
(08:30) Leveraging IoT data for AI applications
(09:31) Improving working conditions of frontline workers with AI
(11:30) Addressing concerns around data privacy and security
(13:10) Feedback and learnings from AI implementations
(15:17) Best practices for AI adoption
(17:16) Role of LLMs
(18:43) Getting started with AI in physical operations
(26:23) Evaluating the quality of your data
(28:03) Learn more and follow up
Transcript:
- [Ryan] Welcome everybody to another episode of the AI For All Podcast. I'm Ryan Chacon. With me today is my co-host, Neil Sahota, the AI advisor to the UN and one of the founders of AI for Good. Neil, how's it going?
- [Neil] Hey, I'm doing well. It's both an exciting and I guess scary time going on with AI in the world right now, but I think we'll get a chance to explore a little bit more of that in our conversation today.
- [Ryan] That's why we do this, Neil. We talk about all the exciting stuff in the AI world so. All right, so today's episode, we're going to talk about how and why physical operations within industries are uniquely positioned to benefit from AI transformation. We're going to talk about AI for frontline workers, best practices in AI adoption.
And to discuss this, we have Evan Welbourne, the Head of AI and Data at Samsara, a company focused on digitizing the world of physical operations. Evan, welcome to the podcast.
- [Evan] Hey, thank you. Great to be here.
- [Ryan] Yeah, it's great to have you. I've been looking forward to this conversation ever since we scheduled it. You have a lot of very exciting things going on at Samsara, both AI and IoT, obviously our other kind of brand is IoT For All, so we're very familiar with what you all do. So glad to have you on talking about AI today. And what I wanted to do is kick things off around physical operations. So in my intro, I mentioned talking about the how and why physical operation industries are uniquely positioned to benefit from AI transformation. But before we jump into that further, could you just high level what physical operations means for our audience?
- [Evan] Yeah, sure. So when we talk about physical operations, we're talking about the kind of the core operations that power the economy through the flow of goods and services. So you can think of things like building infrastructure, logistics, keeping utilities running, and all those kinds of activities that together represent about 40 percent of the US GDP. And then for us, it's especially interesting because those kinds of industries, those operations are typically overlooked when it comes to technology, especially in terms of the more purpose built kinds of solutions that solve some of the complex challenges they face out there in the field.
- [Ryan] And one of the things I know you all have said before is you've talked about connected operations. Why is that, what is that exactly and why is that so top of mind these days?
- [Evan] Yeah, so connected operations is what we're building. You know, we're essentially digitizing the world of physical operations as a way to help our customers digitize their operations, get them in a state where they can be safer, more efficient, and more sustainable.
- [Ryan] So then back to my original question, you know, what makes connected operations, what makes the physical operations space so ripe for AI transformation and the adoption of technologies and solutions, things like that?
- [Evan] Yeah, so there's a few things. So, when it comes to physical operations, a lot of these companies face unique challenges that AI happens to be a good match for. Those are things like having a really large amount of data from the field. It's often difficult to wrangle or to make sense of that kind of data. Time intensive workflows. Often these companies are using like a paper on a clipboard, not even using spreadsheets. So big opportunity there for digitization. And then there's also a lot of risky job environments where the workers have to be constantly vigilant in order to stay safe. So that's like sort of key application areas.
The other point is that across all the things that happen out in the world of physical operations, companies have to deal with this massive petabyte scale data that arrives in the cloud. And then the other point is that like there's actually even more data than that. It's exponentially larger when you get to the data on the edge, like real time video that's being connected, collected on the edge as well as different kinds of sensor data.
And that's a key point that it's beyond the, what we typically think of as multimodal data. It's not just sort of text and images, but it's things like, you know, video, audio, sensor data, things like temperature, inertial sensors, diagnostics from specialized equipment. So all of that together makes for a really complex, very large set of data.
And of course, AI is just a really natural fit for extracting value from that complex mix of data. And so it sort of gets to the point of extracting the valuable insights like a needle from a haystack.
- [Ryan] It sounds like that ability to not only produce and then collect data, but also the complexity of the data makes these industries kind of more unique when it comes to, or when you compare it to other industries with the application or the fit for AI solutions. Would that be a fair statement?
- [Evan] I think so. I mean, it's, there's the multimodal aspect, so there's a lot more different kinds of data at play. And then there's this sort of, the need for more real time assistance, right? So if, if we're going to enact some action in the field or alert workers to, say, an unsafe situation, it's got to be pretty close to real time, which is very different from a situation you often have in the cloud or for like a web based service where really we're just kind of ranking ads behind the scene or something like that. It's just a very different setting.
- [Neil] I don't see a lot of organizations like questioning the value, but I think they wonder, like, incrementally, how much will this help them move the needle? I know there's maybe some institutionalization and the belief that they're, they've done a lot already. So does this move the needle like 1%, 5%? What are you seeing and what's the reality?
- [Evan] Yeah, absolutely. So there's, there's a few examples that come to mind. Some that we're enabling at Samsara and others that are just not directly in line with what we're currently offering, but definitely on the horizon. These are things like AI models for event detection. So that's where we're proactively alerting customers or workers in the field about unsafe behavior. In our offerings, is things like for drivers, you know, we have our dash cams that can alert on inattentive driving. They're distracted while they're driving or that, you know, they're tailgating or, you know, they're using their phone while they're driving. These are all types of event detection that we can bring up an alert on and help the driver sort of correct that behavior in real time. It also helps the safety coaches in the back end to work with the driver to sort of improve over time on those behaviors and then what we found is that that's, you know, in that safety use case, it's really high impact for our customers.
So, for example, if we look back at 2022, across the whole year in aggregate, statistically, we helped prevent about 120,000 crashes, which is really impressive. It's such an important number for us to keep track of. And then there's specific customers like DHL Express that reported a 26 percent reduction in accidents. And then a 49 percent reduction in the costs related to those accidents. That's something that keeps increasing for our customers over time. So there's a lot of examples we get from the field of customers that have both been able to, you know, increase their efficiency but also keep their people safe and, you know, just basically provide a better experience for their workers out in the field.
- [Neil] I mean, that's awesome. Those are some powerful numbers. Are you expecting more widespread adoption? I know we're talking about like commercial use. I mean, where are we going with like individual drivers?
- [Evan] Absolutely. There's many more use cases. And I think with that, we'll see increasingly broad adoption across, we already see adoption across many different industries, as noted from oil and gas to goods and services to, you know, government. And then when we talk about things like fleet, there's even just an expansion there towards things like, you know, preventative or predictive maintenance. That's something we definitely see as possible in this world of physical operations, and that's where we're, you know, we're leveraging trends within the operations data to predict when a piece of equipment or maybe a vehicle is going to need maintenance and do that proactively so that, you know, they can be scheduled ahead of time before it breaks down in the field.
And then another one that's really, you know, it applies to many different kinds of industries, is just automating workflows. So, right, there's a lot of workers that are relying on mobile devices to do these everyday tasks and reports, and then AI can streamline and simplify a lot of those kind of processes. It can automate, you know, completion of a some kind of workflow that happens on the mobile device. And then lastly, it's sort of like doing things like surfacing curated workflows for specific employees.
- [Neil] That's awesome. That's a lot of data, I assume, and I think Ryan will like this, you're leveraging a lot of IoT data.
- [Evan] Yeah, absolutely. I would say it's all IoT data to us, right? So we have text data in the mix, you know, sort of reports and context about what's happening in the customer business. But then out there in the field, it's video, it's audio, it's sensor data, things like, you know, the motion of, of a vehicle or a particular piece of equipment. And then of course it's all of the diagnostics data that come from all of these specialized devices. And that is both huge and complex, and it's also really a rich set of data to get at with machine learning. There's all kinds of really valuable insights there that sort of represent fundamentally new frontier for machine learning, in my view.
- [Ryan] Yeah, we've been very excited to kind of see the, how AI and IoT have worked so well together. If you think about IoT being the ability to collect data from the physical world, and then what do you do with that data, and that's where AI kind of comes in. If I'm listening to this, and I am somebody who works in one of these industries we've already been talking about and showing how AI is going to potentially play a role at a higher level, how do you feel like the adoption of AI solutions within these industries where there are these frontline workers will improve or enhance or even just change their overall working experience? What do you think they will see as the biggest benefits or the biggest changes to their day to day jobs?
- [Evan] The frontline workers are just constantly putting their lives at risk to maintain our infrastructure and frankly just to power the global economy. And so AI is a key technology when it comes to making their jobs easier, more rewarding, and of course safer. So, a couple of the main areas we see in our work are, you know, again, helping prevent that risky behavior in real time, you know, on the edge, we're running models that can do things like, you know, create an in cab audio alert for, you know, the times when the customer is distracted while they're driving or using their mobile phone. And then it lets the, that worker kind of correct their behavior in the moment. And that's invaluable. The other types of key capabilities that we're seeing that really help in the field are, you know, streamline time to insights. You know, AI can automate some of those mundane and time intensive tasks that workers are doing every day. And that's things like, you know, completing forms automatically. It's things like, you know, intelligent search that reduces the time it takes to find some footage or some more information that they're looking for. And then, again, in connection with safety, there's lots of opportunities to take that data from the field in real time and kind of move it offline and then use it for coaching and education and learning development over time so.
- [Neil] You've done a great job articulating a lot of the business value. I think that's fantastic. I would imagine there's probably people in our audience wondering like this, I mean, it sounds great, I get the upside. What about concerns around like data privacy and security, right? I mean, obviously you're tapping into the behavior and using like video, other forms of data. Are there concerns that this, you know, information can be misused?
- [Evan] I think a lot of that comes up when we're talking about the dash cams, the cameras. So we're sort of, we have workers that are on camera at times. And I guess for the most part, what we see with our customers is that they tend to actually welcome the technology. And it's partly because the cameras are there to protect workers and save lives. And, you know, we see that and that's that's recognized by our workers. The other thing that's really powerful and appreciated is that they're tools for exoneration, right? A lot of times, more often than not, our customers are in situations where perhaps there was an accident or a collision, and in fact, they're not at fault, but they don't have any evidence to show that, to exonerate the driver from fault. And so that's really one of the key motivating use cases for our cameras. We can detect crashes, we can record, you know, what actually happened and then our customers can use that immediately.
And so to that end, you know, our cameras are really designed to protect workers and our customers and to help them, you know, not just get home safely at the end of the day but sort of deal with these difficult situations, you know, efficiently and effectively. And then that's that's for the most part what we do hear from from both our customers and the workers in the field.
- [Ryan] What has been the, I guess, reception or feedback that you've received from any of these implementations where I know, you know, putting out the IoT side is the ability to collect the data, but now having the data accessible, what has been the kind of feedback from these frontline workers when they see changes to their day to day, when they see access to this data or, you know, just the general implementation of these solutions that may change the way they just behave and do things on a daily basis?
Has there been any interesting kind of feedback or maybe feedback that's been different than you expected or things you guys have really learned from from these implementations? Because we are still, to a larger population, we still are kind of early in the adoption of AI. So when companies are hearing that, oh, my company is going to bring in these AI solutions, we want them to hopefully feel excited and optimistic about what that means.
So, I'm just curious kind of what the feedback has been like from customers or the employees and the end users of a lot of these solutions.
- [Evan] I would say generally, we have positive to neutral feedback from the people in the field, depending on the situation. In some cases, there are these, if we're talking about event detection, the detectors are there to detect fairly rare kinds of events. They don't happen that often. And so for the worker in the field, it's sort of an occasional alert when it's needed and maybe nothing at all. And then for the sort of statistically for the customer that manages this fleet, it is the big impact because you know, it's spread across many different workers.
But we do, of course, hear stories about, you know, that dash cam, you know, alerted a driver in a way that was allowed them to stop sooner or, you know, enabled them to sort of put their phone down at a time when it was really important and that has made a big difference to them. And then again, as I mentioned, like the exoneration case is something that, for sure, the end workers also appreciate because it exonerates them from fault in those difficult situations.
- [Ryan] You know, we've been talking, I think, a good bit about the applications and the use cases, the feedback, the benefits companies are seeing. From all of the stuff that you all have done, if, for the companies out there listening about or listening to understand how to adopt AI solutions, even on the IoT side, there's elements that could apply here, what are some of the best practices that you would kind of preach to them on how to approach the adoption of AI, let's say LLMs, to achieve, you know, more practical and tangible impact on their business, their employees or customers, just kind of, if we were to start at a high level, what would you kind of, how would you kind of answer that?
- [Evan] Right, it's an important question. I'd say to start with conducting an internal data audit because, of course, really AI runs on data and then it can create this flywheel effect. So if you can conduct an audit of your data, that's gonna help you better understand your business and the most data intensive areas that are surrounding those customer needs that you want to serve.
And then those data intensive areas are where you really want to start with AI and then also the areas where you can probably make the most impact. So there's sort of like assessing your data. What do you have? Where is it? And then there's also an element of data quality and completeness, right? AI is going to need, you know, complete data, higher quality data when you have it. And that's either to set up a model for long term success, or that could be, you know, a lack of data or quality could kind of derail the opportunity for AI.
The other thing I would just quickly point out, there's a need to kind of have a customer feedback loop, I would say, for AI. Most AI solutions, you need to get the customer in the loop to refine and sort of validate that your solution is doing the thing that you want it to do. So if there's a case that you can come to where you've got the data, there's enough data that's clean, and you have an opportunity to present something to the customer and get feedback, that's also just an excellent place to start.
- [Ryan] What about LLMs? So we haven't really talked too much about that. We have in our past conversations, but when it comes to developing solutions for customers, how are you thinking about LLMs? How should they be thinking about LLMs? Like, just kind of, how does that all fit in here?
- [Evan] When it comes to LLMs, I usually like to think about how they can be applied to specific customer needs. And then at the same time, of course, you've got to balance that against what they can do in practice at this point today, and then, you know, what they may be able to do in a couple months. It's, as you know, it's changing really quickly.
And so I think, you know, key places to apply LLMs are, you know, areas where you need some really context rich assistance or you're automating some more mundane administrative tasks that are mostly language oriented. Or on certain kinds of knowledge retrieval, information retrieval that requires searching through a lot of different kinds of documentations.
The thing, with respect to connected operations, I think that LLMs are also a really great enabler for people who are a little bit non expert in the field, and it allows them to interface with technology just using everyday plain language. They don't have to be tech experts. They can just, you know, speak using natural language, or when we talk about multimodal models, they can even use images to sort of communicate with the tech system. And that's also, I think, going to be a great enabler for the world of physical operations.
- [Ryan] So tell me about some of the best practices when it comes to getting started with AI in physical operations. Like what are some of the things that people need to know when it comes to that?
- [Evan] I'd go back to some extent to what I was saying about just understanding where where your needs are, you know, conducting sort of an audit of, you know, problems and opportunities for AI, connecting with a provider like Samsara, who's going to kind of help you understand what kind of products can, you know, help you be more sustainable, help you be more efficient and help your workers, you know, work more safely.
I think another part of it depends on that, you know, physical operations is such a broad set of industries. It's hard to be too general, but I think another part of it is, you know, fleet management. There's all kinds of industries that have fleets and then just kind of having, understanding what your insights are now with your fleet, what, where your gaps are, you know, what more do you need to know? What could really help you? And then, you know, getting a sense of, you know, how much value that will provide to you.
- [Ryan] And how should companies kind of do that? How can they really understand where their gaps are or really, like, is there a kind of a process they can go through?
- [Evan] There's a process that we typically use is just to conduct a pilot. You know, when we're talking with new customers or we're looking, checking if a new product offering is going to work, we'll often conduct a pilot with the customer and that, in that case, we'll share a number of it, say it's for safety, we'll share a number of dash cams and help them get it installed, and then they'll use that for some some number of weeks. And then even in that span of time, we usually see some pretty significant results. They'll understand not just how the technology works, how their customers, how their drivers, say, adapt to the technology, but also, you know, what are the results? You can even see results on that time scale just because there's sort of real time feedback. And then you start to get some of that insight from the field that you didn't have previously, and it's kind of like opening a window towards, you know, what might be possible if you hadn't seen it before.
- [Neil] One of the things we always talk about just generally is if you're going into your first foray in AI is kind of start small, right? And then show some value, build momentum, do more, start scaling. Rather than try to do more of a, you know, big complex type of thing off the bat. What's kind of your approach to trying to figure out, you know, where's the right place to actually get started that small start for like a client?
- [Evan] I think that there is some sort of iteration with data, wherever your starting point is. You know, getting some more data about your business and about your needs and then understanding something about, you know, what value could this technology bring to my company.
And I mean, that's another thing that we partner with customers on is just trying to trying to do things like pilots, trying to sort of uncover for them the types of opportunities they have, and then not only, you know, starting with the pilot and showing sort of like one application, but how could how could it be expanded from there? Like what other kinds of opportunities are there, perhaps sort of leveraging the same kinds of technology and data to not just support safety outcomes but to improve efficiency in various ways.
- [Neil] I think that's a really good, great approach because, you know, I'm reminded back in my early IBM Watson days that after the Jeopardy challenge, everyone's like, what are you going to do with this? And we're trying to figure that out. And, you know, healthcare was a natural fit, a lot of need, a lot of data. And, you know, we were talking about, like, let's do something small, simple, maybe patient intake, you know, kind of streamline some of that process. But, you know, get a lot of pushback from, I won't name who, a lot of different stakeholders saying like, that's not sexy enough, right? Like let's go out and say we're going to cure cancer. And I think there's a lot of expectation that I see out there for businesses that we gotta do something grandiose. Otherwise, why are we doing anything? Is that something you encounter across your work?
- [Evan] It's always a tension, right? I mean, there's not only a hunger for like these big use cases, but, you know, there's the sense that they're possible now because AI has changed so much even in the last year or two. However, that's, you know, the tension is that, you know, we're always trying to have this tight customer feedback. We're working backwards from the applications of the problems that are our customers have today. And in many cases, I think we find that the most useful applications of AI or other technologies are kind of lower hanging fruit. I mean, these are industries that are using a paper and a clipboard. We may, we don't need to deliver the all encompassing, you know, GPT-6 style model for these customers at this phase, that there's a lot more really valuable, low hanging fruit that we can go after if we are in the loop closely with our customers. So I think, like, it's definitely our approach to start small and then kind of expand in these concentric circles around those core use cases that we have with customers.
- [Neil] So it's not necessarily that the latest technology or the newest technology is necessarily the best, right? We're going to create the most value.
- [Evan] Absolutely. And I think we're in a very interesting time, right? Because on the one hand, you know, maybe we can use traditional machine learning or even, you know, deep learning, which used to be the latest thing, to solve a problem, but sometimes, you know, we can use some of the latest technology, some of the foundation models and, you know, for computer vision or for language. And then, you know, I feel that we're at this transitional phase where you can kind of, there has to be a constant question about like which kind of solution do we want to use here, not only to serve our customer, you know, quickly and effectively, but to kind of set both ourselves and our partners up for long term success, right? So if we can find a way to leverage the latest technology, the latest foundation models to deliver solutions, not just effectively, but much more quickly, it's probably a good idea. You know, if we're just distilling, you know, specialized models from a large foundation model, we can do that much more quickly than, you know, the comparatively older way of just building a new, you know, computer vision model for every new use case. So it's definitely a really interesting time to kind of navigate those waters between sort of practical, more classical approaches and like these new opportunities, which seem to be emerging week by week almost.
- [Ryan] That's fantastic. Yeah, this has been a great conversation, Evan. I think there's a lot of interesting things, especially with the space that you all play in, because a lot of times what we've talked to companies in the past about is, you know, they've built an application or solution on the AI side of things, but the data piece is often missing, and I think a big conversation that customers and potential adopters have is, okay, I understand what you can do with my data, but I'm unsure if I have good data, clean data, the right data, if I'm collecting the data the best way possible, or maybe I'm not collecting data at all. How do I get good data, right, in order for these AI, the AI components of a solution to actually be valuable. And I think that's what's an interesting is how the position Samsara is in, in general, is to be able to kind of do both well with a customer. How do companies or how do you suggest companies kind of evaluate the quality of their data as they're listening to this or how they should be thinking about approaching getting better data in order for the AI components to be successful?
- [Evan] I think there's there's a few elements. Of course, it depends on the type of problem that they want to address. And, you know, as I mentioned before, it's always helpful to do a data audit, maybe even a focused one around, you know, they're trying to build a certain solution or what kind of data do we have about it? And then it's also a question of, you know, once you find the gaps in the data, what's gonna sort of plug those gaps? Is it just a, is it a quality problem? Is it something that they can fix by sort of upgrading software? Do they actually need to capture more data that they're not capturing yet? And that's, for example, that's another key place Samsara can often help. And then it's, you know, there's, it's so complex, right? Because in some sense, a solution may be operating on the edge, in which case there's a ton of data, but it can just stay on the edge. In other cases, you know, it's data you really want more centralized. You've got to have it in the cloud. And then there's other kinds of integrations you may need to get the data together. Which is another thing, you know, Samsara has plenty of integrations with partners that, you know, our customers take advantage of. So it's, of course, it's a complex problem, but I would still say kind of start with that audit, understand the problems, understand the gaps, and then, you know, we can work together to find a way to bring it to a place where AI can be an application.
- [Ryan] So for our audience out there who wants to learn more about what you all do and kind of your offerings, follow up with questions, all that kind of good stuff, what's the best way they can do that?
- [Evan] Well, you can always check our website, samsara.com, and, you know, happy to connect with people on LinkedIn. Most of us are there. And, you know, just generally, open to discussions, and, you know, you can also follow our blog is another great place to sort of see what we're up to.
- [Ryan] Well, Evan, we appreciate your time. Neil, any last comments or questions or things from your end before we jump off here?
- [Neil] I think this has been a great discussion, and I really like the fact that even though we're talking about technology, we usually associate that with the digital world, it's good to see a practical application of physical operations, so we really appreciate that, Evan.
- [Evan] Absolutely.
Special Guest
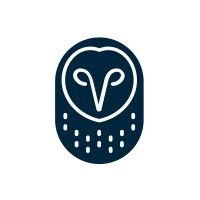
Evan Welbourne
- Head of AI and Data, Samsara
Hosted By
AI For All
Subscribe to Our Podcast

YouTube

Apple Podcasts

Google Podcasts

Spotify

Amazon Music

Overcast











