On this episode of the AI For All Podcast, Raghu Ravinutala, CEO and co-founder of Yellow.ai, joins Ryan Chacon and Neil Sahota to discuss enterprise LLMs and AI in customer support. They talk about the evolution of AI chatbots, the impact on jobs, updating AI systems, saving and increasing costs with AI customer support, industries leading the way, specialized LLMs, using AI for customer insights, and advice for enterprises deciding to adopt AI.
About Raghu Ravinutala
Raghu Ravinutala is the CEO and co-founder of Yellow.ai, a global leader in Conversational AI that delivers autonomous, human-like experiences for customers and employees to accelerate enterprise growth. Since its inception, Raghu and his team have successfully expanded Yellow.ai’s presence across North America, Asia-Pacific, Europe, the Middle East, Africa, and Latin America, with 1100+ customers in 85+ countries.
Interested in connecting with Raghu? Reach out on LinkedIn!
About Yellow.ai
Headquartered in San Mateo, Yellow.ai is a global leader in Conversational AI, delivering autonomous experiences for customers and employees to accelerate enterprise growth. Powered by Dynamic AI agents, the company aims to provide human-like interactions that boost customer satisfaction and increase employee engagement at scale, through its no-code platform.
Key Questions and Topics from This Episode:
(00:34) Introduction to Raghu Ravinutala and Yellow.ai
(00:59) Evolution of AI chatbots
(06:08) Increasing adoption of AI
(08:57) How will AI chatbots impact jobs?
(12:19) What makes companies adopt AI chatbots?
(17:15) Updating AI systems
(20:14) Profiting from AI customer support
(22:05) Industries leading AI customer support adoption
(25:59) Enterprise LLMs and specialized LLMs
(29:51) Advantages of AI chatbots
(31:38) Using AI for customer insights
(35:11) Advice for enterprises deciding to adopt AI
(39:12) Learn more about Yellow.ai
(00:59) Evolution of AI chatbots
(06:08) Increasing adoption of AI
(08:57) How will AI chatbots impact jobs?
(12:19) What makes companies adopt AI chatbots?
(17:15) Updating AI systems
(20:14) Profiting from AI customer support
(22:05) Industries leading AI customer support adoption
(25:59) Enterprise LLMs and specialized LLMs
(29:51) Advantages of AI chatbots
(31:38) Using AI for customer insights
(35:11) Advice for enterprises deciding to adopt AI
(39:12) Learn more about Yellow.ai
Transcript
- [Ryan] Welcome everybody to another episode of the AI For All Podcast. I'm Ryan Chacon. With me is my co-host, Neil Sahota, the AI Advisor to the UN and founder of AI for Good. How are things going, Neil?
- [Neil] Hey, I'm doing all right. I hope everyone out there is enjoying the summer or the winter, depending on what part of the world you're in.
- [Ryan] We also have Nikolai here, our producer.
- [Nikolai] Hello.
- [Ryan] All right. Today's episode, a lot of exciting stuff to talk about. We're going to dive into discussing breaking the barrier when it comes to chatbots, how generative AI can help propel the evolution of conversational interfaces. And to discuss this today, we have Raghu Ravinutala, the CEO and co-founder of Yellow.ai.
They are a conversational AI company focused on delivering autonomous experiences for customers and employees to accelerate enterprise growth. Perfect company to be talking to you about this topic. Raghu, thanks for being on the podcast today.
- [Raghu] Fantastic being here, Ryan, Neil, and Nikolai. Looking forward to a fun conversation.
- [Ryan] Absolutely. So to start things off, let me ask you to talk to us and our audience about comparing chatbots from the, in the past to where they are now and maybe talk a little bit about where they're going with everything going on in the AI space. More high level it for our audience to set the stage.
- [Raghu] Thanks a lot for that introduction, Ryan. And to answer your question, maybe a little bit of context around Yellow.ai as a company itself. We are a company that started in 2016 primarily working towards automating customer support and experience for enterprises. And we've been fortunate to see the entire wave of chatbot evolution right from when Facebook Messenger enabled chatbots on their platform in 2016 to where we are with generative AI. We've worked with over a thousand plus enterprises across several different countries across the world. When the chatbots by their name started in 2016, the primary use case was around information retrieval or providing information back to the users.
And the primary mode of operation was getting the basic keywords from what users were interacting with and fetching the right information and providing the answer back. And then the first level of automation came in with TensorFlow getting open source and getting a a bit of intents and entities and with the basic machine learning, I wouldn't say it's AI, but machine learning was the first technology that was used to find the patterns in the way that users were interacting and getting the right answers for that.
And over a period of time the industry evolved, and I think there were smaller versions of what we called as large language models with the release of BERT, et cetera, in 2018, 2019, that took the large data sets that are available in the internet and without the need for extensive training, you were able to identify intense entities.
And there was dialogue systems being built, not just to answer questions but complete dialogues and complete transactions, be it doing a transaction with the banking system, doing a transaction with airline system and over this period of time, right, from 2016 to 2019, 2020, we have seen a tremendous improvement in the completion rates, deflection rates, and this was resulting in direct ROI impact for our end customers.
And over this time, the number of channels also expanded significantly, moving from Facebook Messenger to WhatsApp, websites, mobile apps, so chatbots were everywhere. They were delivering value, but they were still not up there to the promise that was there when they were considered a universal digital assistant.
And over the last two years, generative AI has taken a leap forward. OpenAI announced ChatGPT. Generative AI was around in a smaller way. I think there were language models with Hugging Face, et cetera, that you're able to get a reasonably high accuracy in terms of understanding and actually generating the answers as well, where it completely reduced the training time, improved the accuracy, improved the deflection rates. But with OpenAI's generative AI, what I think truly changed is the ability to generate human like content and responses. I think that is the biggest difference that we saw with OpenAI. What that enabled is that here though the kind of interactions that were not possible to be automated earlier, be it empathetic responses, negotiating with the users, driving goal based interactions, demonstrating human like empathy. All these were, are now possible with generative AI and and taking it forward. We are seeing improved conversion rates, improved satisfaction rates, and much more longer conversations that humans are having with the latest large language models based chatbots or voice assistants.
So that's the whole loop that we have seen. So we are probably at the level four, level five or level four, level five, at that boundary of chatbot responses.
- [Neil] It's interesting because you talk about like the ability for empathetic engagement, negotiation. Some of these things are actually, they're not new to AI, like artificial empathy has been around for eight, nine years.
But it was always like everything was piecemeal, right? One API over here, another API over there. It was really on companies to string it together to provide this overall experience. Do you feel now with chatbots, all the stuff that's coming bundled together, already self integrated, so it's easier for businesses to use an employee?
Is that the transformation you think has been going on?
- [Raghu] The transformation that's, I think the enterprises are now more ambitious than they were earlier. So the biggest difference before gen AI and now what we are seeing is chatbots and AI systems were seen as supplements to human based support where 70 to 80% of the interactions were handled by humans.
And the chatbots and AI systems were supplementing them to take away some part of the load. Now enterprises are more ambitious. We are in fact, we are talking to our customers and looking at a future, which we call it agent-less customer experience, where companies are looking to drive fully autonomous customer support and experiences, where a lot of the existing customer support personnel would be transformed into AI managers, people who are managing the chatbot system or the AI system, rather than directly answering the calls.
So that is now possible just because the breadth of conversations that now AI is able to handle, be it decision making, be it empathetic responses, be it negotiating, which are not possible, are now suddenly possible.
- [Neil] That's awesome. A little weird tangent for me on this. I'm curious if you're working with any of the airline companies.
There's a guy who's been stranded because of the thunderstorms or issues recently that, thousands of flights cancelled, is that like a ripe opportunity right there?
- [Raghu] That's absolutely a ripe opportunity and typically these situations have been the triggers for a lot of companies to implement automation because that hits them at a time of where a number of consumers are calling them for responses so yeah, absolutely.
We're seeing some of those and hopefully it'll help you, hopefully you don't get stuck in a thunderstorm next time, but in case you get, you'll probably get a resolution faster than before.
- [Ryan] Let me ask you, you mentioned jobs, you mentioned the transition of, if call centers are able to go to be fully autonomous, that those people that were handling the customer interaction will move more to AI management. Do you envision that being something that would require probably a different skill set and different personnel entirely, or is that something that the existing call center team members that were doing this that are now being replaced by the chat bot would be able to be trained and move into that type of role since obviously when it comes to AI, there's always the question of is AI going to take jobs.
So this is a perfect example of taking a something that has been historically done with people and making it autonomous. What happens to those individuals? Is it just, are they going to find jobs elsewhere? Do you think this is something they could adapt and become maybe more in tune with the technology and engineering side to be able to handle the AI pieces?
- [Raghu] The way that I see it, what's happening out there is that it's no doubt that these jobs are going to be impacted. The number of people required for customer support will drastically come down. I'm sure, I think there are areas where companies will position them and make better utilization of that workforce that they have.
If you have seen a recent example, I believe a furniture company has upgraded their contact center agents to be more consultants rather than support providers. So there will be a move towards more higher end interactions that humans are going to handle. But for basic regular customer support, you're going to see a tremendous amount of movement of people away from those roles.
Talking about how these customer support agents are going to move to AI managers, I think there's going to be different skills needed. The skills are more like what you would think of as a contact center trainer or a manager where they are coming up with how the brand needs to respond to customers.
What is their style? What is the the dialogue that they want to be having with their customers? Earlier they were training the recruits into the contact center teams. But now they are going to train the AI agents. They're going to monitor, they were earlier monitoring people on their performance, quality.
Now they've moved towards monitoring the AI systems, their quality, their performance, and managing and correcting the system on the go, which is a different task from just answering the phone calls. There is definitely going to be a change in the job description, what these people are going to do.
But my hope is that with this entire change in technology, people can move on to more upgraded roles and more consulting based roles to their customers than support roles, but there's going to be a massive shift and transformation. That's for sure.
- [Ryan] Do you think that, or I guess, let me ask you this way.
What do you think really needs to happen to make this something that companies feel comfortable adopting? Or are there certain metrics or things that they can see throughout the testing phase in order to feel like this is something they can bring into their business and see good results with the engagement they get with customers?
And the reason I ask is because for me, whenever I jump into a chat bot, the first thing I'm trying to do is talk to a human because going through all the different questions and things to get to an answer feels less efficient than typing in the word human or people or whatever, just talk to somebody so that I can communicate one to one with them.
What do you think companies are going to be looking for when it comes to the decision on how to decide when is the right time for their customer base, as well as when is the technology ready for that experience, especially if they have a wide range of customers from different ages, demographics, languages, and things like that.
What do you think they need to see in order to feel like this is something that I can bring into my business to start seeing real results, both on the bottom line and just with customer experience.
- [Raghu] The reason why contact center and customer support are one of the initial or the first areas that are to be impacted by AI is the reason the, this entire space is very objective.
So the way that the contact center performance is evaluated is very objective. You have first time to response, mean time to resolution, customer satisfaction rates, net promoter score, and as long as, and of course the cost of driving this, as long as these key parameters keep improving with AI being the core provider of the service, companies will start to expand and scale up on these deployments.
From what we have seen so far with our customers, first time to response is unmatchable. You're going to get answers. Hold times are completely eliminated. It's a big move up shift. The second is the mean time to resolution, especially if the task is well defined and have the right integrations. A human would take almost 20 to 30 minutes to go to multiple systems and resolve that query by doing a transaction.
Let's say a reschedule of a flight ticket. Whereas software can get the resolution within a few minutes, we're seeing the trend up quite significantly there. The third is about empathetic responses and customer satisfaction rates. There we have seen a divergence historically where information and transactional use cases where people want to get things done, the AI and chatbots are able to do them much faster and much more cleaner, and we're seeing satisfaction rates much higher.
Where we were seeing an issue was when the user has a complaint or an issue that needs to be emotionally handled, chatbots can become really frustrating. And we have seen human agents do much better, but with the latest technology, we are seeing trends where now the ability to empathetically respond and resolve and even make decisions, for example, you're not happy with some baggage fee. Earlier it required a human to come in and provide an offer, provide a solution for your problem. Now the AI agents can take in the budgets for the day and make real time decisions whether they need to give a discount, they need to waive off, or they need to hold on to the baggage fee, for example.
So now they are taking a little bit more of those tasks. And the initial results show that, I think, they have been effective. But still, in these use cases, the deployment at enterprises have to happen at scale. Information transactions, it's absolutely proven for more emotional and empathetic needs.
We are in the early stages of proving the efficacy of chatbots and AI assistants. The results are very promising right now. My belief is that in the next year or two, we'll see these use cases also deliver higher improvement in metrics across these four different parameters. That's how I look at it, and that's how companies will look at it.
- [Neil] I'm totally with you on that, Raghu. I think the trust factor is a lot better. I don't know if that's just comfort and benchmark results, but I think you alluded to something that seems to be more of a barrier these days, which is scalability. I think a lot of businesses look at this and say, if I have a new offering, or I run frequent promotions, how much work do I have to do then to keep the AI updated to provide the quality of service?
What do you think about that? What do you see on the scalability side?
- [Raghu] Companies need to invest resources in keeping the AI updated. Unlike traditional applications like mobile apps and websites, which could be static, the information that the company wants to convey to the customers and interact with the customers keep changing and keep metamorphosizing day in and day out.
Let me take the example of your situation itself, Neil, when you probably got stuck in a flight. That's not static information. So there was something that happened during those 2, 3 days that resulted in your complaint or your reach out to the company and that information of what needs to be answered, it needs to be trained and provided back to the AI system. It's an imperative for companies like us to enable our customers and enterprises to have all the tools, all the management systems to keep the AI system updated, make changes, and monitor the quality of responses and keep on fine tuning that.
But it also requires them to invest resources to manage this. So the equation that the companies would be looking at is, I'm going to take additional investment in buying the software and dedicating certain set of people to manage this. Whether this investment justifies itself for the overall automation and the cost reductions that I'm seeing from a regular contact center perspective, and that is the ROI calculations that companies are doing as they are evaluating this technology. And I think we are right now converging on two mega trends. One is we are going to be in a high interest rate scenario for the next three, five, who knows, some people are predicting probably this decade as well where I think the indexing on efficiency is super high and that is coming together with where AI technology is available to be deployed at scale.
So these forces are made for each other at some point of time where it is, AI is going to really helping enterprises address the number one problem that they have, and I think companies will start to move really fast in adopting this.
- [Neil] It's a really important point to say this is an investment. It's not static, or you have to maintain it.
You have to keep the teaching, everything up to date. But I think you also make a really powerful point around the inflation, right? Because you got me thinking about like wage inflation and how McDonald's is now replacing people that take your order in the drive thru with AI bots, right? It's not just a cost savings move, they've now seen, again, benchmark results, improved order accuracy, and the ability to actually take more changes to a, your hamburger, chicken sandwich, whatever you're ordering and capture that appropriately. So there's a level of improved customer satisfaction they're also experiencing.
- [Raghu] We are seeing more and more of that for sure.
And another trend that we are seeing in line with what you're saying, Neil, is historically the front office has been, front office when I'm in customer support, marketing, sales, they've all been siloed due to several reasons because of expertise of people, their different budgets, et cetera.
And with more and more of AI systems, the end user doesn't see the company as three different silos. They are talking to the brand and companies are seeing this opportunity of using the AI front end as a unifying force across the different silos of the front office of company. Imagine going through a drive thru center where you're not just ordering, but you're probably dissolving some of your concerns about the takeaway or the delivery. So it's a unified interface that can support, but also can sell. So it not just becomes a cost center, but it can also become a profit center. So we are seeing that trend happen as well.
- [Ryan] Do your see or from your experience engaging with different types of companies across different industries certain industries more prime to adopt these chatbots and technologies when it, with AI or do you or and are there others that may be, we may not be there yet for what you envision them really needing to see value?
- [Raghu] Yes. We do see certain industries adopting this technology faster. The key characteristics of a company or industry that is fast in adopting automation in front office is one where they are spending a significantly high cost on customer interactions.
There are a very high number of interactions that their contact centers are having with their customers. Paid financial services, retail, utilities, telecom, these are the number one. Second is a lot of these companies where their core services are reasonably commoditized. Where if you see banking, insurance, there are financial products that everyone provides.
They differentiate with customer experience. So I think these are the companies that we see adopting it faster. Third, where companies with reasonably lesser regulatory impact or regulatory concerns to deal with. Potentially pharma would be a little bit more hesitant towards it, whereas companies related to retail and financial services,
I think they are more open. So as a summary of this, the companies that we are seeing with a significantly faster adoption is retail, financial services, utilities, and telecommunications.
- [Neil] What about the hospitality industry? Are you seeing anything there?
- [Raghu] Absolutely. So we have some of the large hospitality brands as our customers.
So we're seeing hospitality also take on let's say airlines and resorts, et cetera. So we see that happen. The consideration there for hospitality is that the, while the customer service is something that they're looking to automate, we're still seeing that there is a relationship building over long term for sales and et cetera.
So where there is certain amount of human based experience that they are prioritizing as well.
- [Neil] Anywhere there's high touch, that's an opportunity.
- [Raghu] Let me give an example there where it's a really large resort brand in the United States. This is the conundrum that a lot of hospitality companies are facing is they have a single number for people to contact either for sales or customer support.
Finally what they're seeing is that 80% of their human costs right now is going for customer support, whereas 20% is going for sales. And they want to actually change that, reverse or flip that equation where a lot of their human resources go into consulting and providing the experience and selling, which is revenue accretive.
And a lot of support to be automated and beat transactions. So reduce the costs and redeploy the cost more on the revenue generation side and where a real human interaction can drive up the consulting sales and the experience of the end customer.
- [Ryan] I wanted to ask you about the behind the scenes piece of a lot of this, and I've had some conversations external from this podcast about companies focusing on building their own LLMs and kind of these more specialized LLMs for similar tooling for them internally as well as externally, but can you talk to our audience about what a specialized LLM is, how that works, what that means in relation to maybe how other people are thinking about LLMs more in the public space?
- [Raghu] So you have these foundational models which are OpenAI GPT-4, Anthropic, and Cohere, which are trained on this 170 billion or close to a trillion parameters that just have the entire learning of the universe, universal data on the language. There is, there are a few problems that come with directly using these LLMs to drive conversations.
One, these LLMs are generic. They're trained to provide data on all language in the universe, whereas enterprises want controlled interaction with their end customers that is related to their products and services, control of data and APIs and transactions. The second is they also need configurability.
Every brand has their own style and way of managing the interactions, and they want to be able to configure precisely how these dialogues need to be managed. The third is about security and managing their own customers and data. Again, public LLMs, you're sending out data to these external LLMs, et cetera, which is causing a concern around security and privacy.
The fourth is they're only using them for a narrow set of use cases. And the broad LLMs are not as effective when dealing with this core set of narrow use cases because they are optimized generically. So where specialized LLMs are coming in is taking some of the low end versions or smaller versions of the really large language models, which are still large, let's say a GPT-2.5, et cetera, and specifically training for enterprise specific use cases. We recently launched a paper around how to manage and control the data and systems that they are exposed to. So these models are fine tuned towards specific use cases trained with specific data and systems, and they are managed within the SAS ecosystem of companies like us.
So we have taken some of these models, incorporated as a part of our dialogue system, trained them with core enterprise data and built tools to configure and manage how these systems interact with their end users. And that is what is delivering significant value to the enterprises, where you're able to get the insane smartness from the large language models, but adding the capabilities of configurability, controllability, security and them being deterministic in providing the enterprise use cases and responses back to the customers. This is how we see the direction of how LLMs will be adapted for enterprises.
- [Nikolai] You use the word deterministic. This is something I find interesting because like previously, to get a person, like a human employee to reliably, they have to, you have to train them to follow a particular protocol and when someone calls them for support, they may or may not follow the protocol strictly.
Maybe they're tired. Maybe they're frustrated with something, but like with a, with these chatbots, with AI, it's completely deterministic and people always want to feel like, they want to feel like their problem is being solved. They don't want to get a thought in their head that is the person on the other end of the line really solving my problem, are they actually invested.
But I think that's probably part of the reason why the satisfaction has gone up. It's entirely deterministic, like the AI is doing exactly that, and it's 100% focused on that.
- [Raghu] A hundred percent. And the other part is also that a lot of the human interactions earlier were not really digitized in the sense that you can't go back and check whether everyone is even following the protocol or not. Now with AI, all of these are digitized, and you have the ability to go determine what is the right way of responding to the customer, look at outcomes and changing at a system level rather than at a user or person level.
The customer support and experience is more now managed systemically rather than dependent on a person or process and adherence per se.
- [Neil] So I think there's some interesting untapped value here, right? Because especially with the big brands, they have their own language and other things going on.
But I probably can't say who it was, but a major hotel chain was actually looking at something to tap into audio data. This was late 2019. Don't get me started on audio data, big untapped data source that AI can exploit that we can't as much. But one of the things they were actually doing was thinking about how they can improve customer service.
And they realized that they had some people out in the field that are probably providing excellent customer service, but it's never really captured. And they were experimenting with a pilot to see could they capture that, use AI to identify what some of those best practice moments are, what is this top performer doing to trigger the top performance, capture that, flag it, and see if we can make that a best practice that you can teach everyone to try and uplift your entire customer service experience. Because of COVID and hotels obviously shutting down, all those things that kind of got, I think, lost in the shuffle, but given the work you're doing, like with Yellow.ai and customer service, for certain industries, would that be a future point of value that could you not just do all these things and providing the service and the brand, but could the interactions of these AI systems yield some better best practices?
- [Raghu] Absolutely. So let me take an example of a beverage brand that provided an AI assistance to end customers to create recipes for cocktails and mocktails, etc.
So what the AI system was able to show to the brand back was there's a certain section or geographies where the consumers preferred spicier cocktails and there was a region or segment of customers that preferred more sweeter cocktails. And this kind of provided feedback to their, to the brand on their marketing strategy, product strategy on the right things to do that are super personalized for these segment of users.
Historically the amount of data that's exchanged in contact centers, there are about 400 billion calls right now made every single year, very few insights to the brand on what they need to change from their product, from their marketing, from their segmentation. All that data was not available because these were all analog, not configured, unlabeled data that was happening out there.
With AI, that entire data is now labeled, managed, and it's already starting to show signs where this can provide deep insights into not just the consumer, but like how they can change their brand, their positioning, their marketing, their product line which is highly invaluable for a lot of these companies. And we see those, we see that happening, Neil, absolutely.
- [Ryan] One of the last things I wanna ask you before we wrap up here is as people are listening to this in the enterprise, commercial space and looking to explore adopting generative AI tools, LLMs into their business in some different way, anything related to these AI solutions, how do you recommend they think through that adoption process?
Because there are some situations, and this is again comes from conversations I've had external to this, about companies looking to maybe build their own versus adopt an existing solution. So how do you advise companies to think about that decision when it comes to bringing these types of tools and solutions into their business to see the benefits across their enterprise for either internal or external reasons?
- [Raghu] Historically, when a new technology is is introduced, and especially over the last one, two decades there have been tools that enable companies to build the initial set of features, and that's where open source come into play, where historically companies have the ability to build applications on their own, companies have an ability to build mobile apps and systems on their own, and I think that's great initially while proving out the concepts and proving out the efficacy of the technology. But most of the time, the enterprises end up to use third party or vendor based solutions for production deployment because the vendor, vendors, they are building, let's say, a focused solution towards entire customer support. Getting an interaction automated with technologies, I'm sure you can take out of the box, open source, configure a little bit and manage it, but the entire workflow that needs to go along with it, be it power, managing the conversations, driving others, connecting the humans, tools to update the content, fine tuning the models, driving configurability, the enterprises by themselves either would need to spend significant amounts of capital to develop that without the necessary back or ROI in the outcomes, whereas they could use a specialized vendor like Yellow.ai in, who have already built all these systems, tested across several set of enterprises and pay as a subscription cost and derive the value because this is not the core of a lot of companies that that do as their core purpose.
They are companies that are selling retail products, providing great healthcare, providing great banking services, focus on their core, and this is something that they can use off the shelf, out of the market that best in class companies have built their entire infrastructure to solve a customer problem than just provide AI frameworks that need to be configured, developed within the enterprise.
That's how we see it. In the initial days, you'll always see companies trying out things till the market consolidates a little bit, but the end case is always about using the best in class solutions.
- [Ryan] Neil, Nikolai, any last comments, questions before we wrap up?
- [Neil] I think this has been a great discussion, and I think Raghu, you've done a great job of showing the powerful value propositions that are associated with using AI to help automate and run or manage customer service.
- [Raghu] It's been a fantastic discussion, Ryan, Neil, and Nikolai. Fantastic questions and looking forward to many more in the future.
- [Ryan] Absolutely. For our audience who wants to learn more about what you have going on at Yellow.ai or reach out, follow up, anything regarding this discussion, what's the best way they could do that?
- [Raghu] Best way is our website, yellow.ai. And even better way is that we have an AI assistant out there. I think that's, we eat our own dog food, so website and our digital assistant out there are probably the best ways to get more information.
- [Ryan] Fantastic. Well, Raghu, thank you so much for taking the time.
Excited to get this out to our audience and have you and the company showcased out to the world and what you have going on. As we continue to build more new content, love to have you back and involved as much as possible to keep sharing that expertise to our audience.
- [Raghu] Thank you. Thank you very much everyone. Have a great day ahead.
Special Guest
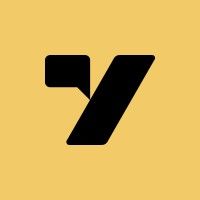
Raghu Ravinutala
- Co-Founder and CEO, Yellow.ai
Hosted By
AI For All
Subscribe to Our Podcast

YouTube

Apple Podcasts

Google Podcasts

Spotify

Amazon Music

Overcast











